Counting Nests of Shags With YOLO To Assess the Wellbeing of the Antarctic Ecosystem
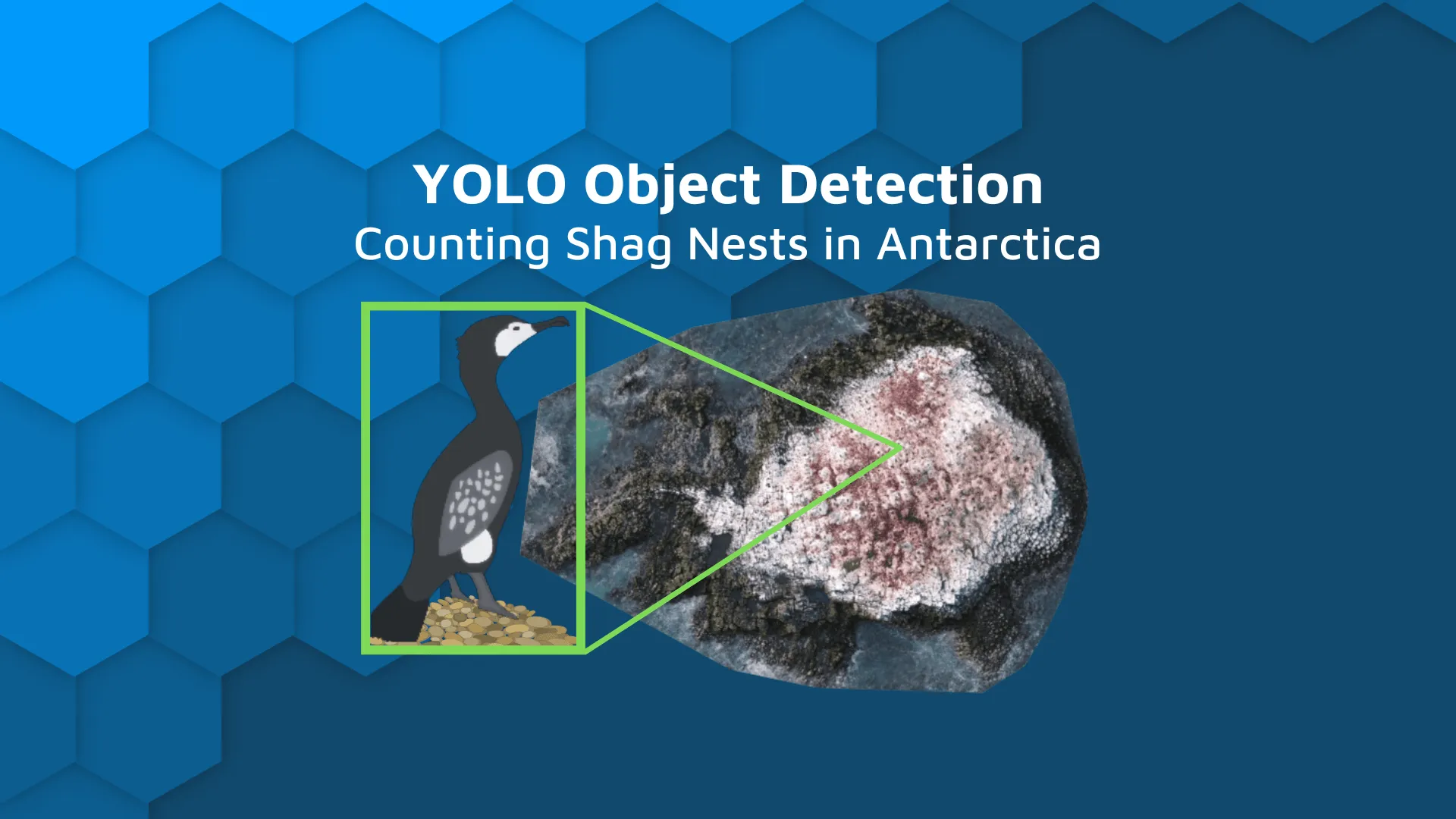
<a href="https://appsilon.com/ai-research/" target="_blank" rel="noopener">Appsilon's ML Team</a> recently partnered with ecologists at <a href="https://arctowski.aq/en/about-station/" target="_blank" rel="nofollow noopener">the Arctowski Polish Antarctic Station</a> managed by <a href="https://ibb.edu.pl/en/laboratory/robert-bialik/" target="_blank" rel="nofollow noopener">IBB PAS</a>. The scientists here are focusing on monitoring the Antarctic ecosystem, flying drones to capture images of the precious polar wildlife. There are many possible fronts on which we hope to collaborate in the future. Still, to start the collaboration, we have decided to test if computer vision techniques can be used to automate the counting of cormorant (shag) nests in drone imagery. <img class="wp-image-15903 size-full" src="https://webflow-prod-assets.s3.amazonaws.com/6525256482c9e9a06c7a9d3c%2F65b2ac1d749d9aa8bef9eee5_1-5.webp" alt="Image 1 - An overview of the Arctowski station - a year round research facility in the Antarctic, run by IBB PAS (Photo by Marek Figielski)" width="1280" height="401" /> Image 1 - An overview of the Arctowski station - a year-round research facility in the Antarctic, run by IBB PAS (Photo by Marek Figielski) <i>This article was co-authored by Appsilon's ML Lead <a href="https://appsilon.com/author/jedrzej/" target="_blank" rel="noopener">Jędrzej Świeżewski</a> and Katarzyna Fudala from <a href="https://ibb.edu.pl/en/laboratory/robert-bialik/" target="_blank" rel="nofollow noopener">IBB PAS</a>.</i> Table of contents: <ul><li><a href="#introduction">What are Antarctic Shags?</a></li><li><a href="#the-importance">Why are Shags (Cormorants) Important in Antarctica?</a></li><li><a href="#why-drones">Why use Drones for Antarctic Shag Ecological Surveys?</a></li><li><a href="#identifying-birds">Identifying Antarctic Birds - (Not Always) Black and White</a></li><li><a href="#identifying-nests">Identifying Nests of Antarctic Birds</a></li><li><a href="#machine-learning">Antarctic Bird Nests and Object Detection - A Case for Machine Learning</a></li><li><a href="#yolo">Applying YOLO Object Detection: A Custom Measure of Success</a></li><li><a href="#highlights">Highlights of the Results</a></li><li><a href="#summary">Summary</a></li></ul> <hr /> <h2 id="introduction">What are Antarctic Shags?</h2> The Antarctic shag (Leucocarbo bransfieldensis) is the southernmost of the world's cormorant species and its population (< 12,000 pairs) is restricted to the Antarctic Peninsula, the South Shetland archipelago, and Elephant Island. <img class="size-full wp-image-15905" src="https://webflow-prod-assets.s3.amazonaws.com/6525256482c9e9a06c7a9d3c%2F65b01d0899f2296f27b4a3a5_2.webp" alt="Image 2 - Antarctic shag colony on the Shag Rock island, picture courtesy of Department of Antarctic Biology, IBB PAS" width="1999" height="1500" /> Image 2 - Antarctic shag colony on the Shag Rock island, picture courtesy of Department of Antarctic Biology, IBB PAS It is the only flying bird of the Antarctic Peninsula region that predates near-exclusively on fish, requiring plunge diving to the bottom near the shore; thus, the bird occupies the niche of a major predator of demersal fish. <h3>Just how deep can Cormorants dive?</h3> The maximum diving depth and duration for the Antarctic shag is <a href="https://www.cambridge.org/core/journals/antarctic-science/article/abs/shags-in-antarctica-their-feeding-behaviour-and-ecological-role-in-the-marine-food-web/A77516977BCEB9020E734D1E3A26E414" target="_blank" rel="nofollow noopener">truly impressive</a>. When hunting for fish they can submerge more than 110 m deep and spend five and a half minutes diving! It is the deepest and longest-duration record for any flying bird in Antarctica. <h2 id="the-importance">Why are Shags (Cormorants) Important in Antarctica?</h2> In the Southern Ocean surrounding Antarctica, several unique species (e.g. the Patagonian toothfish or krill) are harvested in commercial fisheries managed by the Convention for the Conservation of Antarctic Marine Living Resources (<a href="https://www.ccamlr.org/en/organisation/home-page" target="_blank" rel="nofollow noopener">CCAMLR</a>). For the fishery to serve its purpose it has to be managed sustainably. Sustainable fishing means leaving enough fish in the ocean so that they can survive and reproduce to provide enough food for other animals that depend on them. The CCAMLR sets limits to determine how much can be harvested each year to minimize the negative impact on the ecosystem. To decide how much fish can be taken out of the ocean without disrupting the population, scientists estimate how much biomass of any particular species there is. The problem is, we cannot simply count fish in the ocean so the population sizes are estimated based on careful monitoring and interpretation of the data arising from it. The monitoring is focused on a few selected indicator species, which are most likely to respond to changes in the ecosystem. Cormorants are one of these indicator species, making them vital to monitoring shifts in the ecosystem. <h3>What makes Antarctic shags a good indicator species?</h3> Following the decline of seal and whale populations prior to 1970, demersal fish stocks were depleted in the South Shetland Islands by industrial fishing during the 70s to early 80s. Since 1982, the fisheries are regulated by CCAMLR and many were closed due to overexploitation. At the same time, a marked decline in the Antarctic shag breeding population has been recorded. The undeniable link between the condition of the shag population and its prey makes it the only non-krill-dependent species on the list of CCAMLR indicators. Antarctic shags' diet, as well as, e.g., the size of the breeding population are used to monitor changes in coastal fish population and enrich the biomonitoring of living marine resources. <h2 id="why-drones">Why use Drones for Antarctic Shag Ecological Surveys?</h2> Scientists from the Department of Antarctic Biology, IBB PAS are using drones to survey the size of the Antarctic shag population, which, as prof. Robert Bialik, Head of the Department, points out, "<i>is a more accurate method, less invasive for the animals and does not require human presence in the colony area</i>". However, counting cormorant nests in aerial photographs is not a simple task. Antarctic shags very often nest in mixed colonies with chinstrap penguins, requiring a lot of effort and patience to find their nests among hundreds or even thousands of penguin nests. <h3>Remotely sensed imagery and computer vision</h3> Katarzyna Fudala, an ecological monitoring specialist at the Department of Antarctic Biology, emphasized that adapting computer vision techniques capable of automatically detecting cormorant nests would be a significant improvement for scientists involved in Antarctic shags research based on aerial photogrammetry. An efficient object detection model could not only save scientists' time, but as shown below, could find nests the scientists miss. Sound science is vital for improving our understanding of the Antarctic ecosystem and for sustainable management of living marine resources. <h2 id="identifying-birds">Identifying Antarctic Birds - (Not Always) Black and White</h2> The difference in morphology between shags and penguins seems obvious. <img class="size-full wp-image-15907" src="https://webflow-prod-assets.s3.amazonaws.com/6525256482c9e9a06c7a9d3c%2F65b01d083a40c80f90229a19_3.webp" alt="Image 3 - Morphology difference between shags and penguins" width="1317" height="1999" /> Image 3 - Morphology difference between shags and penguins But when we look at both species from a distance... <img class="size-full wp-image-15909" src="https://webflow-prod-assets.s3.amazonaws.com/6525256482c9e9a06c7a9d3c%2F65b01d09099015d6184d0d42_4.webp" alt="Image 4 - Morphology difference from a distance" width="1999" height="1336" /> Image 4 - Morphology difference from a distance And in particular from a bird's-eye view (or, to be more precise, from a drone's-flight perspective)... <img class="size-full wp-image-15911" src="https://webflow-prod-assets.s3.amazonaws.com/6525256482c9e9a06c7a9d3c%2F65b2ac4af99b81a7b8ed9f55_5.webp" alt="Image 5 - Morphology difference from a drone's flight perspective" width="1999" height="1414" /> Image 5 - Morphology difference from a drone's flight perspective The obvious differences appear to be blurring and the ubiquitous black and white come to the fore- the colors being part of the adult plumage of both species. <h3>Why are Antarctic birds black and white?</h3> The black and white plumage of these birds is not an evolutionary accident and is related to the ocean-dependent lifestyle of both species - birds use countershading to camouflage themselves from potential predators. When seen from below, a white belly blends in with light-filled surface waters while from above a black back merges with the dark abyss of the ocean. <h2 id="identifying-nests">Identifying Nests of Antarctic Birds</h2> Fortunately, the obvious difference in the nest styles of the two species is particularly evident in aerial images. Antarctic shags build impressive nest structures from algae and feathers fused with feces, while chinstrap penguin nests consist of small pebbles painstakingly collected by their owners before breeding. However, nest recognition is also not straightforward - a shag colony, especially one mixed with penguins, undergoes a number of visual transformations during the season related to breeding phenology (figure below): incubation (a), presence of chicks in the nests (b) or fledglings scattered around the nest (c). Moreover, the nest and its surroundings look very different during the breeding (a-c) and in the post-breeding (d) season, and the level of difficulty in identifying nests on orthophotos depends on a number of factors such as the presence of snow or lighting conditions. <img class="size-full wp-image-15913" src="https://webflow-prod-assets.s3.amazonaws.com/6525256482c9e9a06c7a9d3c%2F65b2ac4bcd5bc8264be12705_6.webp" alt="Image 6 - Colony visual transformations during the season" width="1999" height="1414" /> Image 6 - Colony visual transformations during the season <h2 id="machine-learning">Antarctic Bird Nests and Object Detection - A Case for Machine Learning</h2> Rather quickly into the discussions we realized that the data collected and used by the scientists could be transformed in a way ideal for training object detection models. We found several key factors which made this a good case to study for machine learning. If you're wondering if machine learning could be applied in your research see if the following also apply to your case: <ol><li>There were multiple images available, spanning all the seasons and phases of the lifecycle of shags.</li><li>Images came from three distinct locations - perfectly suited for splitting the data geographically, ensuring and assessing the robustness of our models.</li><li>The images were of very high spatial resolution (ranging from ~6mm per pixel to 2.5cm per pixel). These are resolutions 10-50x better than the best satellite imagery currently available to the public!</li><li>Each image was geolocated, which allowed us to study the nests and performance of our models over time.</li><li>The individual nests of the cormorants were marked and geolocated. With a rather constant physical size (roughly 0.5m in diameter), the point markings could easily be translated into bounding boxes.</li></ol> <img class="wp-image-16174 size-full" src="https://webflow-prod-assets.s3.amazonaws.com/6525256482c9e9a06c7a9d3c%2F65b01d0cfb45e346e0b201cf_antarctic-shag-nests-zoom-in-from-drone-imagery.gif" alt="One of the islands hosting a colony of Antarctic shags and a colony of chinstrap penguins. Image courtesy of Department of Antarctic Biology, IBB PAS" width="894" height="642" /> One of the islands hosts a colony of Antarctic shags and a colony of chinstrap penguins. Image courtesy of Department of Antarctic Biology, IBB PAS <h2 id="yolo">Applying YOLO Object Detection: A Custom Measure of Success</h2> We formulated the machine learning problem to be a case of object detection as it seemed the latest installments of the <a href="https://appsilon.com/object-detection-yolo-algorithm/" target="_blank" rel="noopener">YOLO family of models</a>, such as YOLOv6 would work well on the data. However, in this case, we weren't so much interested in the precise sizes of the nests but rather in the presence or absence of them. So, we developed a custom way of assessing the detections based on the confidence of the prediction and the level of overlap with the ground truth. The metric is defined as follows: <ul><li>We accept only predictions with a confidence score above a certain fixed threshold.</li><li>We look at predictions that overlap with a nest above a certain threshold of their area</li><li>If a given nest is covered by predictions above a certain threshold of its area we count this as a True Positive - a correct detection of a nest (this is important as we tile the large maps both for the training of models and inference).</li><li>Nests not covered enough are counted as missed - False Negatives.</li><li>Predictions not aligned enough with any nest are counted as False Positives.</li></ul> <h2 id="highlights">Highlights of the Results</h2> Several approaches could be considered, but we have decided to try one of the most demanding for any model. We picked a single image from a relatively large colony of shags (having ~250 nests) and trained an object detection model on it. Using a few neat tricks on the way we got the model to perform very well on the training colony. <img class="size-full wp-image-15917" src="https://webflow-prod-assets.s3.amazonaws.com/6525256482c9e9a06c7a9d3c%2F65b7d34b5591af1433a8d311_4f9aebaa_8-2.webp" alt="Image 8 - An Antarctic island as seen from a drone. Nests of cormorants are marked with blue dots, the predictions of our model are marked with green boxes. The predictions are very good" width="1336" height="901" /> Image 8 - An Antarctic island as seen from a drone. Nests of cormorants are marked with blue dots, the predictions of our model are marked with green boxes. The predictions are very good Clearly, the predictions only appeared within the colony, e.g., no penguin nests were misidentified as shag nests. Several nests were missed, and in a few cases, the model saw nests that were not initially marked by experts. In fact, some of such cases were reconsidered and led to a correction of the labels! <blockquote><i>"We are impressed with the results and high quality that Appsilon was able to produce in a small amount of time. Appsilon's ML technique has great potential to shift the way we monitor Antarctic fauna in the future."</i> -- <b>Robert Bialik</b>, Head of Antarctic Biology Department, Prof. of Institute of Biochemistry and Biophysics PAS</blockquote> <img class="size-full wp-image-15919" src="https://webflow-prod-assets.s3.amazonaws.com/6525256482c9e9a06c7a9d3c%2F65b2ac4d1be4c3cacfed202f_9.webp" alt="Image 9 - Close-ups of the above image, highlighting how challenging a task the model had to face" width="1531" height="703" /> Image 9 - Close-ups of the above image, highlighting how challenging a task the model had to face The examples show many correct predictions, as well as, both a missed nest (left) and individual predictions of nests not initially labeled as such (both). The real test came when we applied the model trained on a single image from a single colony to images of colonies kilometers away from the first (20 and 40 kilometers to be exact): <img class="size-full wp-image-15921" src="https://webflow-prod-assets.s3.amazonaws.com/6525256482c9e9a06c7a9d3c%2F65b7d34cff233005820f8c3c_f1818bd4_10-1.webp" alt="Image 10 - The results on data never seen by the model, showing very good performance" width="1163" height="720" /> Image 10 - The results on data never seen by the model, showing very good performance Notice the correctly identified cormorant nest which lies within the penguin colony - in the lower part of the middle section of the image. <img class="size-full wp-image-15923" src="https://webflow-prod-assets.s3.amazonaws.com/6525256482c9e9a06c7a9d3c%2F65b7d34de407a924913e2f6b_132d8d34_11-1.webp" alt="Image 11 - Good performance of the model on unseen data - another example" width="727" height="662" /> Image 11 - Good performance of the model on unseen data - another example <hr /> <h2 id="summary">Summary of Counting Nests of Antarctic Birds with YOLO</h2> In this project, we combined unique and important research data with the latest object detection techniques. Already the first iterations of the effort led to very promising results, potentially shifting the way monitoring Antarctic fauna is perceived in the ecological community and opening a new and important door to an improved understanding of the ecosystem of the Antarctic. A paper explaining the details of the method, quantifying the results, and exploring their limitations is in preparation. Last but not least, this project was run as part of the <a href="https://appsilon.com/data-for-good/" target="_blank" rel="noopener">Data for Good</a> program at Appsilon. Want to see how AI, computer vision, and machine learning models can help your projects? <a href="https://appsilon.com/data-for-good/#contact" target="_blank" rel="noopener">Reach out to us</a> to learn more about computer vision and machine learning solutions and discuss a possible collaboration!