Unraveling the Segment Anything Model (SAM)
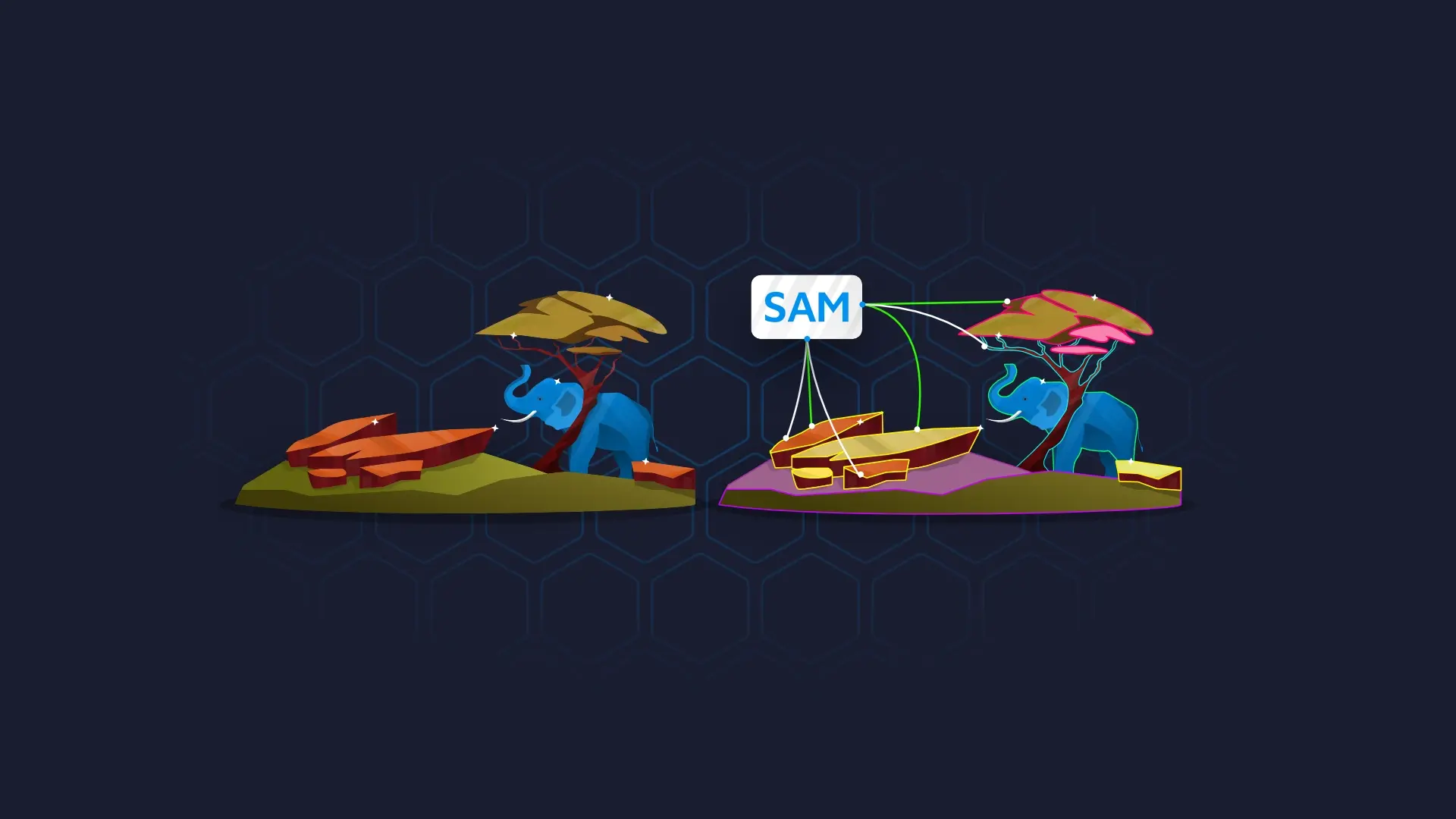
SAM, the Segment Anything Model, was recently released by Meta's FAIR lab. It is a state-of-the-art image segmentation model aiming to revolutionize the field of computer vision. SAM is built on foundation models that have significantly impacted natural language processing (NLP) and focuses on promptable segmentation tasks, adapting to diverse downstream segmentation problems using prompt engineering. In practical applications, SAM can segment objects by simply clicking or interactively selecting points to include or exclude from the object. It can identify and generate masks for all objects present in an image automatically, and after precomputing the image embeddings, SAM can provide a segmentation mask for any prompt instantly, enabling real-time interaction with the model.
<img class="aligncenter size-full wp-image-21224" src="https://webflow-prod-assets.s3.amazonaws.com/6525256482c9e9a06c7a9d3c%2F65b019ef3519a56046b49aa8_image-segmentation-model-SAM-from-Metas-FAIR-lab.webp" alt="image segmentation model SAM from Meta's FAIR lab" width="1000" height="202" />
<h2>Wildlife Data Image Segmentation with SAM</h2>
The wildlife research landscape is intricately linked with effective image analysis. SAM, with its pioneering "segment-anything" philosophy, transforms this space by effortlessly tackling diverse wildlife datasets. Camera traps, pivotal in wildlife monitoring, amass vast collections of images. Traditional analysis struggles with such scale, but SAM, in its versatile approach, identifies and isolates fauna with ease. This can not only accelerate conservation measures but also showcases SAM's potential in broader applications, from aerial wildlife surveys to granular behavioral studies, reinforcing its revolutionary impact on contemporary wildlife research.
<img class="size-full wp-image-21222" src="https://webflow-prod-assets.s3.amazonaws.com/6525256482c9e9a06c7a9d3c%2F65b019f0b6f7f96ac6a5fcca_image-segmentation-segment-anything-model-of-wildlife.webp" alt="image segmentation - segment anything model of wildlife" width="800" height="218" /> Original image couresty of ANPN/Panthera
When prompted by clicks, SAM effectively eliminated foreground elements, ensuring the segmented representation was solely focused on the intended subject, be it an animal or any other point of interest. For most samples, it was able to discern between animal subjects and their environment using only a single click, demonstrating an uncanny knack for the given task.
<img class="size-full wp-image-21252" src="https://webflow-prod-assets.s3.amazonaws.com/6525256482c9e9a06c7a9d3c%2F65b019f29ca976faa7d4bc61_segment-anything-model-segmenting-wildlife-in-camera-traps.webp" alt="segment anything model segmenting wildlife in camera traps" width="800" height="266" /> Original image couresty of ANPN/Panthera
One of the facets of SAM that stood out was its efficiency in segmenting animals when presented with bounding boxes. This is a remarkable feature as the boxes provided were not closely tailored specifically for accurate segmentation. Yet, SAM handled them deftly, offering clear cut-outs of the animals from their environment.
<img class="size-full wp-image-21250" src="https://webflow-prod-assets.s3.amazonaws.com/6525256482c9e9a06c7a9d3c%2F65b019f3b4b56ca016be5840_segment-anything-model-segmenting-wildlife-in-camera-traps-gorillas-and-pangolins.webp" alt="segment anything model segmenting wildlife in camera traps gorillas and pangolins" width="800" height="263" /> Original image couresty of ANPN/Panthera
While both prompting techniques - clicking and bounding box - are distinct in their approach, the resulting segmentations turned out to be of comparable quality. This flexibility in choice of segmentation method without compromising on the outcome quality is a testament to SAM's robustness.
<img class="size-full wp-image-21256" src="https://webflow-prod-assets.s3.amazonaws.com/6525256482c9e9a06c7a9d3c%2F65b019f5b4b56ca016be5a2d_segmenting-elephants-from-wildlife-camera-background-different-positioning.webp" alt="segmenting elephants from wildlife camera background different positioning" width="800" height="271" /> Original image couresty of ANPN/Panthera
<img class="size-full wp-image-21258" src="https://webflow-prod-assets.s3.amazonaws.com/6525256482c9e9a06c7a9d3c%2F65b019f5b6f7f96ac6a5ffce_segmenting-elephants-from-wildlife-camera-background.webp" alt="segmenting elephants from wildlife camera background" width="800" height="308" /> Original image couresty of ANPN/Panthera
SAM's ability to distinguish individual entities within a crowd is laudable. Whether provided with practically crafted bounding boxes or prompted with a mere one or two clicks, the model discerned between multiple entities with finesse. This capability is particularly crucial in wildlife contexts, where discerning individual animals within a group is often required.
<img class="size-full wp-image-21260" src="https://webflow-prod-assets.s3.amazonaws.com/6525256482c9e9a06c7a9d3c%2F65b019f6d0b1df80ab6cc7be_segmenting-individual-animals-with-Metas-FAIR-lab-segment-anything-model.webp" alt="segmenting individual animals with Meta's FAIR lab segment anything model" width="800" height="441" /> Original image couresty of ANPN/Panthera
Venturing into the realm of segmenting specific body parts of animals was slightly unpredictable. The model's performance varied across different body parts, and while it wasn't always clear which parts SAM would excel at, the results were satisfactory for the parts it did manage to segment.
<img class="size-full wp-image-21262" src="https://webflow-prod-assets.s3.amazonaws.com/6525256482c9e9a06c7a9d3c%2F65b019f74367c4d0119069cc_using-ai-to-segment-animal-body-parts-in-camera-traps.webp" alt="using ai to segment animal body parts in camera traps" width="800" height="613" /> Original image couresty of ANPN/Panthera
No model is without its challenges, and SAM is no exception. While its accomplishments in the wildlife domain are commendable, there are areas awaiting refinement. A closer examination of some samples reveals minor discrepancies in boundary precision. For instance, subtle details like the right ear or half of a tusk were occasionally overlooked. These nuances, while minor, underscore the potential avenues for further fine-tuning and enhancement.
<img class="size-full wp-image-21232" src="https://webflow-prod-assets.s3.amazonaws.com/6525256482c9e9a06c7a9d3c%2F65b019f89ca976faa7d4c11b_precision-discrepencies-with-the-segment-anything-model-SAM.webp" alt="precision discrepencies with the segment anything model SAM" width="800" height="601" /> Original image couresty of ANPN/Panthera
<h2>Plankton Data / Microorganism Image Segmentation with SAM</h2>
In the following section we’ll analyze how well SAM performs in lipid-sac segmentation tasks on binocular copepod data.
To effectively segment regions in plankton data, unlike wildlife images, SAM requires the plankton bodies to be vertically or horizontally aligned, samples with clear and discernible boundaries, and carefully crafted bounding boxes.
<img class="size-full wp-image-21264" src="https://webflow-prod-assets.s3.amazonaws.com/6525256482c9e9a06c7a9d3c%2F65b019f9fa26a4961d7777d0_using-SAM-segment-anything-model-on-lipid-sac-segmentation-tasks.webp" alt="using SAM - segment anything model on lipid-sac segmentation tasks" width="870" height="316" /> Image from <em><a href="https://appsilon.com/copepod-prosome-and-lipid-sac-segmentation-with-machine-learning/" target="_blank" rel="noopener">Machine Learning and Plankton: Copepod Prosome and Lipid Sac Segmentation</a></em> (Appsilon)
In more realistic cases, SAM completely fails to segment lipid sacs via bounding boxes.
<img class="size-full wp-image-21244" src="https://webflow-prod-assets.s3.amazonaws.com/6525256482c9e9a06c7a9d3c%2F65b019fb9ca976faa7d4c248_segment-anything-model-SAM-failure-to-segement-body-parts-via-bounding-boxes.webp" alt="segment anything model SAM failure to segement body parts via bounding boxes" width="763" height="273" /> Image from <em><a href="https://appsilon.com/copepod-prosome-and-lipid-sac-segmentation-with-machine-learning/" target="_blank" rel="noopener">Machine Learning and Plankton: Copepod Prosome and Lipid Sac Segmentation</a></em> (Appsilon)
Interactive segmentation, necessitating between 2-6 clicks, emerges as a preferable method. In this approach, SAM's segmentation capabilities manifest more consistently, delivering trustworthy results.
<img class="size-full wp-image-21230" src="https://webflow-prod-assets.s3.amazonaws.com/6525256482c9e9a06c7a9d3c%2F65b019fc9d0ea11e186fc700_Metas-FAIR-lab-SAM-better-suited-for-identifying-microscopic-organisms-for-surroundings.webp" alt="Meta's FAIR lab SAM better suited for identifying microscopic organisms for surroundings" width="800" height="614" /> Image from <em><a href="https://appsilon.com/copepod-prosome-and-lipid-sac-segmentation-with-machine-learning/" target="_blank" rel="noopener">Machine Learning and Plankton: Copepod Prosome and Lipid Sac Segmentation</a></em> (Appsilon)
Zooming out to a broader perspective, SAM might be better suited for distinguishing entire microscopic organisms from their surroundings. Segregating subcellular components, like lipid sacs, prosomes or antenna poses a more significant challenge, suggesting SAM's potential value lies in broader organism-level segmentation in such microscopic settings.
<img class="size-full wp-image-21242" src="https://webflow-prod-assets.s3.amazonaws.com/6525256482c9e9a06c7a9d3c%2F65b019fdf9568bdc75308f26_segment-anything-model-SAM-better-for-organism-level-segmentation.webp" alt="segment anything model SAM better for organism-level segmentation" width="724" height="262" /> Image from <em><a href="https://appsilon.com/copepod-prosome-and-lipid-sac-segmentation-with-machine-learning/" target="_blank" rel="noopener">Machine Learning and Plankton: Copepod Prosome and Lipid Sac Segmentation</a></em> (Appsilon)
<h2>Medical Data Image Segmentation with SAM</h2>
SAM's performance in medical image segmentation (MIS) is a mixed bag. MIS is challenging due to complex modalities, fine anatomical structures, uncertain and complex object boundaries, varying optical quality of imagery and wide-range object scales. Despite these challenges, SAM has shown promising results in some specific objects and modalities. However, it has also failed in others, indicating that its zero-shot segmentation capability may not be sufficient for direct application to MIS. SAM performs better with manual hints like points and boxes for object perception in medical images, leading to better performance in prompt mode compared to everything mode. As expected, SAM seems to perform much better in easier tasks such as organ segmentation than more real world use cases such as tumor segmentation. Though the model performed decently in easier tasks, it failed miserably in more realistic cases.
In such cases the model was able to segment out the organ / bone with realistic bounding boxes OR up to 6 clicks.
<img class="aligncenter size-full wp-image-21266" src="https://webflow-prod-assets.s3.amazonaws.com/6525256482c9e9a06c7a9d3c%2F65b019fd4367c4d011906f6d_using-SAM-segment-anything-model-for-medical-data.webp" alt="using SAM segment anything model for medical data" width="800" height="303" />
SAM's proficiency wanes when tasked with tumors. While it can discern tumors, it often renders them as indistinct blobs, failing to capture the nuanced boundaries or the extended tendrils typical of malignant growths. This limitation persists even with color-enhanced or ostensibly simpler samples.
<img class="aligncenter size-full wp-image-21248" src="https://webflow-prod-assets.s3.amazonaws.com/6525256482c9e9a06c7a9d3c%2F65b019feaac7a284a0ecadb9_segment-anything-model-SAM-struggles-to-segment-tumors.webp" alt="segment anything model SAM struggles to segment tumors" width="379" height="162" />
<img class="aligncenter size-full wp-image-21246" src="https://webflow-prod-assets.s3.amazonaws.com/6525256482c9e9a06c7a9d3c%2F65b01a002c0171ee6e599582_segment-anything-model-SAM-struggles-to-identify-tumors-with-color-enhanced-images.webp" alt="segment anything model SAM struggles to identify tumors with color-enhanced images" width="726" height="739" />
On particularly simple or straightforward samples, SAM demonstrated consistent performance. Its segmentation was predictable when dealing with these less complex medical images.
<img class="aligncenter size-full wp-image-21236" src="https://webflow-prod-assets.s3.amazonaws.com/6525256482c9e9a06c7a9d3c%2F65b01a02299b8d6d8425e2aa_SAM-demonstrates-consistent-segmentation-performance-in-less-complex-medical-images.webp" alt="SAM demonstrates consistent segmentation performance in less complex medical images" width="800" height="388" />
As anticipated, SAM is helpless when faced with intricate or non-standard samples. However, there's a silver lining: aiding SAM with preprocessed images or additional assistance can sometimes coax out better results, albeit inconsistently.
<img class="wp-image-21254 size-full" src="https://webflow-prod-assets.s3.amazonaws.com/6525256482c9e9a06c7a9d3c%2F65b01a0258ad7af22dfdff11_segment-anything-model-struggles-with-non-standard-samples.webp" alt="segment anything model struggles with non-standard samples" width="675" height="439" /> Difficult segmentation task
<img class="wp-image-21220 size-full" src="https://webflow-prod-assets.s3.amazonaws.com/6525256482c9e9a06c7a9d3c%2F65b01a04e64b1cc59a11c370_difficult-task-for-SAM-segmentation.webp" alt="difficult task for SAM segmentation" width="787" height="531" /> SAM result on difficult task
<img class="wp-image-21226 size-full" src="https://webflow-prod-assets.s3.amazonaws.com/6525256482c9e9a06c7a9d3c%2F65b01a05e31daa8c65fc1211_improving-sam-segmentation-model-with-zoom.webp" alt="improving sam segmentation model with zoom" width="769" height="376" /> Assisted sample (zoomed in)
<img class="size-full wp-image-21234" src="https://webflow-prod-assets.s3.amazonaws.com/6525256482c9e9a06c7a9d3c%2F65b01a06588d6f7ecdfd024e_results-on-assisted-SAM-segmentation.webp" alt="results on assisted SAM segmentation" width="763" height="370" /> Results on assisted sample
For samples where even a non-expert human eye would find it challenging to discern a tumor, SAM unsurprisingly struggled to achieve any level of segmentation whatsoever.
<img class="aligncenter size-full wp-image-21238" src="https://webflow-prod-assets.s3.amazonaws.com/6525256482c9e9a06c7a9d3c%2F65b01a076ee3169213c5b912_SAM-struggles-to-surpass-human-eye-segmentation.webp" alt="SAM struggles to surpass human eye segmentation" width="800" height="252" />
<h3>Extensions</h3>
We explored MedSAM and SAMhq, two notable offshoots of the original SAM model. MedSAM, crafted with medical imaging in mind, and SAMhq, aiming to perfect segmentation, certainly sparked interest in many corners. While their foundational concepts and demonstrations suggest potential, our hands-on evaluation, primarily using medical data, revealed a different story. MedSAM often required meticulously crafted bounding boxes and generally underperformed SAM in our tests. SAMhq, on the other hand, displayed slight improvements on intricate boundaries but still seemed not quite ready for real-world medical deployments. Perhaps refining these models on specific datasets might sacrifice some broad applicability but could lead to much-needed performance gains.
<h4>MedSAM</h4>
<img class="aligncenter size-full wp-image-21228" src="https://webflow-prod-assets.s3.amazonaws.com/6525256482c9e9a06c7a9d3c%2F65b01a09d29d4bcb96835f40_MedSAM-medical-image-segmentation-performance.webp" alt="MedSAM medical image segmentation performance" width="800" height="324" />
Tailored for the medical imaging domain, MedSAM, rooted in SAM's ViT-Base backbone, was developed using a comprehensive dataset of over one million image-mask pairs, representing various imaging modalities and cancer types. While their foundational concepts and demonstrations suggest potential, our hands-on evaluation, primarily using medical data, revealed a different story. MedSAM often required meticulously crafted bounding boxes and generally underperformed SAM in our tests.
<h4>SAM-hq</h4>
<img class="aligncenter size-full wp-image-21240" src="https://webflow-prod-assets.s3.amazonaws.com/6525256482c9e9a06c7a9d3c%2F65b01a0ae872295044989609_SAMhq-medical-image-segmentation-performance.webp" alt="SAMhq medical image segmentation performance" width="800" height="318" />
Created to address SAM's perceived shortcomings in segmenting intricately structured objects, SAMhq introduces the High-Quality Output Token, aiming to elevate the mask prediction quality. Drawing from a relatively small but fine-grained dataset of 44k masks, SAMhq promises improved segmentation quality. In our own evaluations, SAMhq displayed marginal improvements on intricate boundaries but still seemed not quite ready for real-world medical deployments.
Note that these advancements underscore the intricate balance between specialization, generalization, and the resulting performance. While both MedSAM and SAMhq were built on SAM's robust foundation, their efficacy in practical scenarios varies. The subtle enhancements seen with SAMhq and the precise demands of MedSAM provide valuable insights into the complexities of fine-tuning foundational models for specific domains or refined outputs. Perhaps a little more fine-tuning on niche datasets might help give up some level of generalizability for a necessary improvement in performance.
<h2>Discussion of SAM's Efficacy</h2>
Within the realm of medical imaging, SAM demonstrates clear limitations. While capable in handling simpler tasks, its struggles become evident in more complex scenarios, such as distinguishing intricate tumor boundaries. Especially in cases where even human interpretation is challenged, SAM's segmentation falls short. Its current state suggests that careful discretion is needed when considering its use in medical image segmentation, underscoring the importance of domain-specific tools for such critical applications.
While SAM introduces a transformative approach to instant segmentation with its ability to intuitively "cut out" any subject, MBAZA offers a complementary strength in rapid biodiversity monitoring with AI, even in offline settings. The fusion of SAM's wide-ranging adaptability with MBAZA's efficient classification may herald a new era in wildlife data processing.
In the microscopic realm, SAM presents a promising tool for distinguishing microorganisms from their milieu, particularly benefiting environmental and medical studies. While its strength lies in broad segmentation, it falls short in isolating intricate sub-cellular details. This gap is where more sophisticated plankton segmentation solutions, adept at segmenting lipid sacs and prosomes, could complement SAM. By combining SAM's broad organism-level differentiation with another solution’s detailed sub-cellular analysis, researchers could achieve a more comprehensive and efficient microscopic image analysis, thus broadening application possibilities in diverse fields.
SAM's "Segment Anything" philosophy signals a transformative step in image analysis. While its expansive capabilities are pioneering, the real-world implications across domains like wildlife, microscopic studies, and medical imaging present both opportunities and challenges. SAM's versatility, its potential synergies with other technologies, and areas where it may fall short, offer a holistic view of its promise and limitations.
You can check out SAM for yourself using the demo at: <a href="https://segment-anything.com/demo">https://segment-anything.com/demo</a> .