How to Load SAS Files in R: Transitioning from SAS to R with Seamless Data Integration
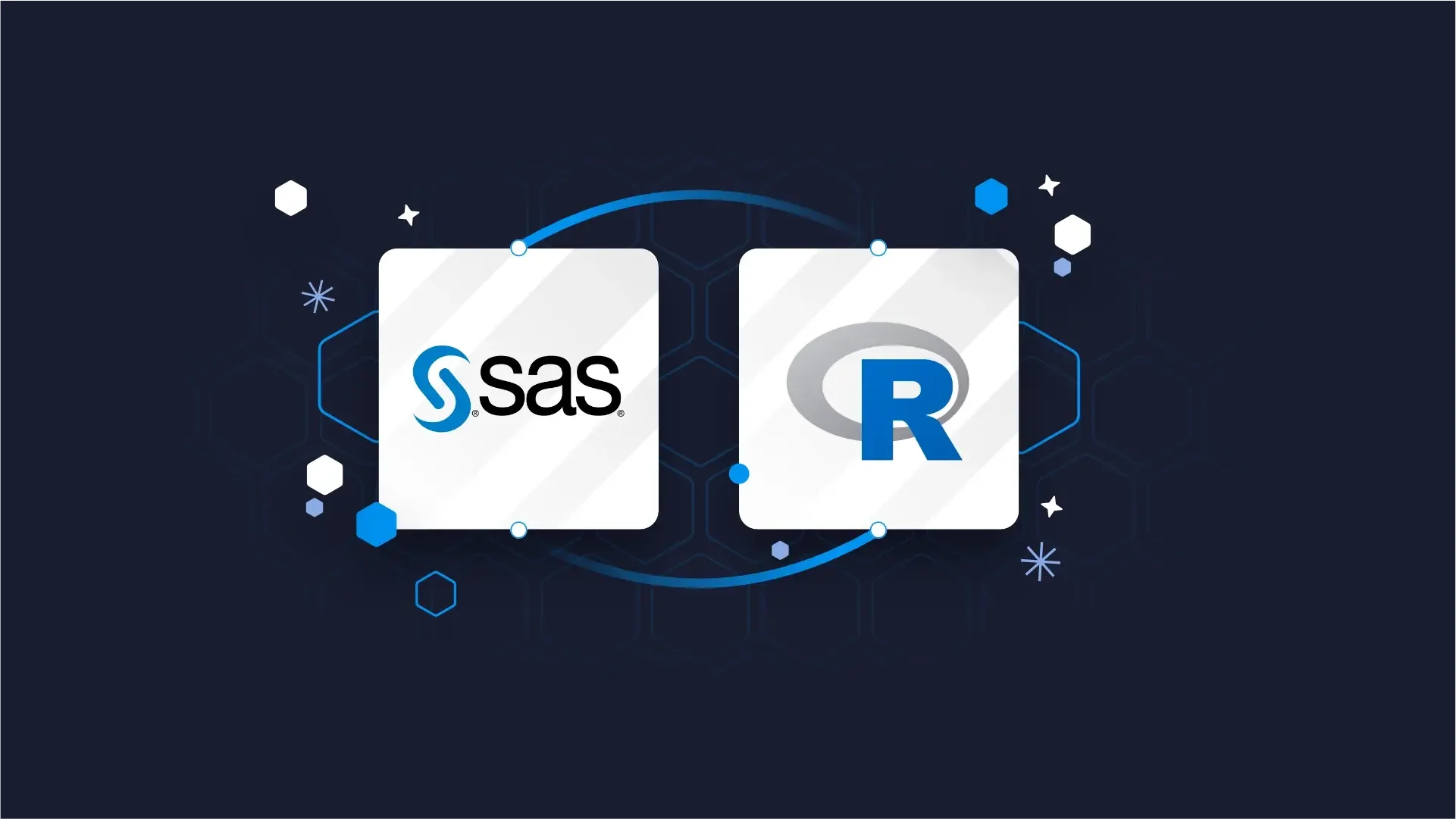
Using R as an alternative to SAS (Statistical Analysis System) <a href="https://appsilon.com/sas-vs-r-programming/" target="_blank" rel="noopener">offers bespoke interactivity on top of R routines</a>. It enables <b>effective technical handling </b>while <b>engaging non-technical users</b> through interactive data storytelling. Transitioning from SAS to R can be a challenge for many data analysts and programmers. But the solution is within reach. It can be easy if part of your SAS pipeline produces data that you use to create reports from R. In this article, we will explore <b>how to integrate SAS data into your R workflow</b>, allowing you to harness the strengths of both tools. We will focus on reading and writing SAS data files in R and overcoming common challenges. By the end of this guide, you'll be well-equipped to bridge the gap between SAS and R, making your data analysis journey smooth and efficient. <h3>TL;DR:</h3> <ul><li style="font-weight: 400;" aria-level="1">Transitioning from SAS to R offers enhanced technical functionality, making data interaction more intuitive. </li><li style="font-weight: 400;" aria-level="1">Explore how to <b>smoothly transition</b> and <b>integrate </b>data from SAS and R.</li><li style="font-weight: 400;" aria-level="1">Understand SAS File Types: <ul><li style="font-weight: 400;" aria-level="2"><b>Data Files (.sas7bdat) - </b> hold tabular data similar to R dataframes</li><li style="font-weight: 400;" aria-level="2"><b>Catalog Files (.sas7bcat)</b> - contain dataset metadata</li></ul> </li> <li style="font-weight: 400;" aria-level="1"><b>Read SAS Data in R</b> and<b> Write SAS Data from R</b> using the <b>haven </b>package with practical examples.</li> <li style="font-weight: 400;" aria-level="1">Best Practices: <ul><li style="font-weight: 400;" aria-level="2">Prioritize reproducibility</li><li style="font-weight: 400;" aria-level="2">Use targets pipeline for routine tasks</li><li style="font-weight: 400;" aria-level="2">Seek guidance (from R/SAS communities and platforms like Stack Overflow)</li></ul> </li> <li style="font-weight: 400;" aria-level="1">The haven package simplifies SAS and R data interoperability.</li> </ul> <h3>Table of Contents</h3> <ul><li><a href="#understanding">Understanding Different SAS File Types</a></li><li><a href="#writing">How-To: Writing SAS data from R</a></li><li><a href="#best-practices">Best Practices</a></li><li><a href="#conclusion">Conclusion</a></li></ul> <h2 id="understanding">Understanding Different SAS File Types</h2> SAS has many <a href="https://support.sas.com/resources/papers/proceedings/proceedings/sugi27/p069-27.pdf" target="_blank" rel="noopener noreferrer">types of file objects</a>. We will explore how to use R to both read and write the following types of SAS objects: <ol><li style="list-style-type: none;"><ol><li><b>Data Files (.sas7bdat):</b> These files store tabular data, including numeric, character, and date variables. SAS data files are the most common type and similar to R data frames.</li><li><b>Catalog Files (.sas7bcat):</b> Catalog files contain metadata about datasets, including variable formats, labels, and other attributes.</li></ol> </li> </ol> <h2 id="reading">How-To: Reading SAS data</h2> To read SAS files in R, we can use the {<a href="https://haven.tidyverse.org/" target="_blank" rel="noopener noreferrer">haven</a>} package, created and maintained by <a href="https://www.tidyverse.org/" target="_blank" rel="noopener noreferrer">the tidyverse ecosystem</a>. It provides functions to read SAS datasets. Here's a step-by-step guide to reading SAS files in R: <pre><code> #install.packages(“haven”) library(haven) <br>sas_data <- read_sas("file.sas7bdat") </code></pre> You can use this approach both for .sas7bdat and .sas7bcat extension files. <h3>Encoding Issues</h3> SAS datasets might not use standard encodings. To handle these issues, specify the encoding when using read_sas(): <code>read_sas("file.sas7bdat", encoding = "UTF-8")</code> <h3>Dealing with SAS Labels</h3> In R, we handle value labels by using factors. However, SAS does it in a different way (<a href="https://haven.tidyverse.org/articles/semantics.html" target="_blank" rel="noopener noreferrer">semantics from SAS</a>). Haven provides the labeled S3 classes to allow importing labeled vectors into R. From the documentation vignette, it showcases an example on how it can deal with labelled SAS object files. <pre><code> x1 <- labelled( sample(1:5), c(Good = 1, Bad = 5) ) <br>x2 <- labelled( c("M", "F", "F", "F", "M"), c(Male = "M", Female = "F") ) <br>tibble::data_frame(x1, x2, z = 1:5)</code></pre> <img class="size-full wp-image-21446" src="https://webflow-prod-assets.s3.amazonaws.com/6525256482c9e9a06c7a9d3c%2F65b019cd5a8828d69797f006_image_2023-10-26_10-25-52.webp" alt="A code snippet displaying two labeled vectors in R. The first vector, x1, contains integers with labels "Good" for the value 1 and "Bad" for the value 5. The second vector, x2, contains characters with labels "Male" for the value M and "Female" for the value F." width="276" height="241" /> Labeled Vectors in R Code Snippet <img class="size-full wp-image-21450" src="https://webflow-prod-assets.s3.amazonaws.com/6525256482c9e9a06c7a9d3c%2F65b019cf431bfa246f4f1184_image_2023-10-26_10-29-00.webp" alt="A code snippet displaying a tibble (a type of data frame in R) with three columns: x1, x2, and z. The x1 column contains integers with associated labels, such as "Good" for the value 1 and "Bad" for the value 5. The x2 column contains character values labeled as "Male" or "Female". The z column contains consecutive integers from 1 to 5." width="325" height="141" /> Tibble Data Frame in R <h2 id="writing">How-To: Writing SAS data from R</h2> To write SAS data from R, you can also use the haven package: <pre><code> my_data <- data.frame( ID = 1:5, Name = c("Bob", "Ed", "Rod", "Dav", "Eva"), Value = c(90, 85, 78, 92, 88) ) <br> write_xpt( my_data, path = "output_file.sas7bdat" ) </code></pre> <h3>Missing values</h3> Newer version of haven already deals with missing values in the same format as “NA” from R. You can also specify a missing value manually if required by using tagged_na(). <pre><code> my_data <- data.frame( ID = 1:5, Name = c("Bob", "Ed", "Rod", "Dav", "Eva"), Value = c(90, 85, 78, 92, 88), na_values = tagged_na("Not applicable") ) <br>write_xpt( my_data, path = “output_file.sas7bdat" ) <br> read_sas("output_file.sas7bdat”) </code></pre> <img class="size-full wp-image-21452" src="https://webflow-prod-assets.s3.amazonaws.com/6525256482c9e9a06c7a9d3c%2F65b019cfb4b56ca016be4269_image_2023-10-26_10-47-21.webp" alt="A code snippet showcasing a tibble (a specialized data frame in R) with four columns: ID, Name, Value, and na_values. The tibble lists 5 rows of data, where each row corresponds to a unique individual with an associated name, a numerical value, and an NA value in the na_values column." width="292" height="122" /> Tibble Data Display with Individual Records in R <h2>Example</h2> Let’s dive in a simple example using SAS datasets. For this scenario, we’ll download the CARS dataset from SASHELP library. <img class="size-full wp-image-21454" src="https://webflow-prod-assets.s3.amazonaws.com/6525256482c9e9a06c7a9d3c%2F65b019d003aa52514f96cb0a_image_2023-10-26_10-52-15.webp" alt="A split-screen interface from SAS Studio, displaying server files and folders on the left and an output data table on the right. " width="975" height="422" /> SAS Studio <h3>Set library to sas data file</h3> Since SASHELP is a library dataset, it’s not in a SAS data file (sas7bdat). This means that we must save it to the proper format before downloading the data file. To do this, just run the SAS program: <pre><code> %Let username = your_username; Libname out "/home/&username/sasuser.v94/"; Data out.cars_data; set sashelp.cars; run; </code></pre> Remember to update your folder username variable without parentheses. Now you can download the data file and use it in R. <img class="size-full wp-image-21456" src="https://webflow-prod-assets.s3.amazonaws.com/6525256482c9e9a06c7a9d3c%2F65b019d2eeb66a4943210408_image_2023-10-26_11-00-38.webp" alt="A close-up view of SAS Studio. The panel on the left shows a directory with the files "cars_data.sas7bdat" and "save to data files.sas." On the right, there's a context menu with options such as "Open," "New," "View File as Text," and a highlighted "Download File" option." width="720" height="753" /> SAS Studio <h3>Playing with data – Summary statistics</h3> To illustrate the example in R, let’s calculate a summary statistic of all columns by the column Type. In SAS, this can be done with the utility helper “Summary statistics”. <img class="size-full wp-image-21458" src="https://webflow-prod-assets.s3.amazonaws.com/6525256482c9e9a06c7a9d3c%2F65b019d320852b3d3e133e33_image_2023-10-26_11-05-44.webp" alt="Statistical Results for the "SASHELPCARS" dataset: It is a detailed table presenting statistical results for different car types, including metrics like "Mean," "Std Dev," "Minimum," and "Quartile Range."" width="975" height="400" /> Statistical Results for the "SASHELPCARS" dataset You can print the result as a pdf file It also returns the code: <pre><code> ods noproctitle; ods graphics / imagemap=on; <br>proc means data=SASHELP.CARS chartype mean std min max median n nmiss vardef=df qrange qmethod=os; var MSRP Invoice EngineSize Cylinders Horsepower MPG_City MPG_Highway Weight Wheelbase Length; class Type; run; </code></pre> In R, a similar approach that results in a pdf file can be done with the package summarytools. <pre><code> library(haven) library(dplyr) library(summarytools) <br>data <- read_sas("cars_data.sas7bdat") grouped_data <- data %>% group_by(Type) <br>view(dfSummary(grouped_data)) <br></code></pre> <img class="size-full wp-image-21460" src="https://webflow-prod-assets.s3.amazonaws.com/6525256482c9e9a06c7a9d3c%2F65b019d52700d7cf10b1aa3e_image_2023-10-26_11-14-18.webp" alt="A split interface on SAS Studio displaying summaries of two data frames." width="1110" height="421" /> Comparative summary of two datasets: 'Hybrid' cars on the left and 'SUV' cars on the right. If you desire to have the result in a dataframe, just update the code: <pre><code> summarised_data <- data %>% group_by(Type) %>% summarise( across( where(is.numeric), list( mean = mean, stdev = sd, median = median, min = min, max = max, iqr = ~IQR(..1, na.rm = TRUE) ) ) ) </code></pre> <img class="size-full wp-image-21513" src="https://webflow-prod-assets.s3.amazonaws.com/6525256482c9e9a06c7a9d3c%2F65b019d6b556fdf5a54d5d9a_ezgif.com-gif-maker-12.webp" alt="A dark-themed interface displaying the summarised data from the file "read_write_sass.R"" width="975" height="285" /> Snapshot of the summarized data Now all we have to do is save the dataframe to SAS data files. <pre><code> write_xpt(summarised_data, "cars_summarised.sas7bdat") </code></pre> You can upload the file back to SAS. <img class="size-full wp-image-21517" src="https://webflow-prod-assets.s3.amazonaws.com/6525256482c9e9a06c7a9d3c%2F65b019d810698cdbd6bc95ca_ezgif.com-gif-maker-13.webp" alt="SAS interface is displayed highlighting the process of uploading files." width="939" height="534" /> Upload the file back to SAS <h2 id="best-practices">Best Practices</h2><ol><li><b>Reproducibility:</b> You can document the steps you take when reading and writing SAS data with R Markdown or <a href="https://appsilon.com/tag/quarto/" target="_blank" rel="noopener">Quarto</a>. This is an important <a href="https://nceas.github.io/sasap-training/materials/reproducible_research_in_r_fairbanks/" target="_blank" rel="noopener noreferrer">aspect of reproducibility</a>.</li></ol> If you desire to run the workflow on a routine, then you can consider using <a href="https://appsilon.com/r-targets-reproducible-data-science-pipeline/" target="_blank" rel="noopener">targets pipeline</a>. <ol start="2"><li><b>Seek Help:</b> If you require further guidance, don't hesitate to seek help from the <a href="https://community.rstudio.com/" target="_blank" rel="noopener noreferrer">R community</a> or <a href="https://communities.sas.com/" target="_blank" rel="noopener noreferrer">SAS communities</a>. Collaboration can often lead to quicker solutions.</li></ol> Also, Stack Overflow is a great resource, and it’s quite possible that someone has already faced and shared a solution similar to yours. <h2 id="conclusion">Conclusion</h2> Using the haven package to read and write SAS data has eased out much of the struggles in SAS and R interoperability. This guide showcases how to read SAS files and deal with common issues related to that process. Do you want to get more out of your data with custom analytics and solutions? <a href="https://appsilon.com/#contact" target="_blank" rel="noopener">We're here to help</a>.