Beyond SAS: How R is Revolutionizing Pharma and Life Sciences
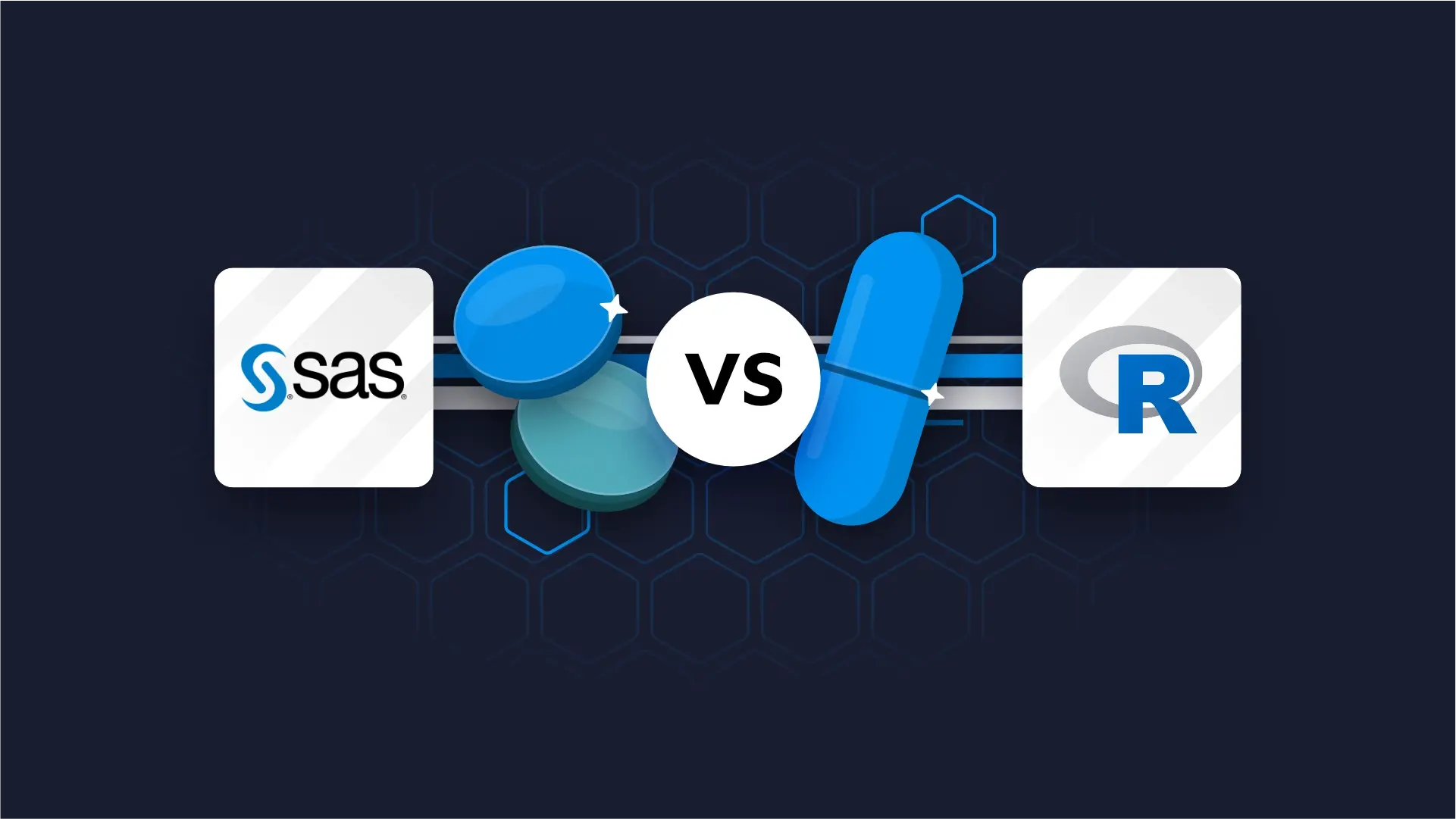
Statistical analysis and data handling play a pivotal role in the life sciences and pharmaceutical industries. The need for accurate and robust data analysis cannot be overstated, as it underpins critical decisions related to drug development, clinical trials, and patient outcomes. In this context, selecting the right software for data analysis and visualization is paramount. One long-standing debate in these sectors revolves around choosing between SAS and R as the preferred tool for these tasks.
SAS (Statistical Analysis System) and R are two prominent software options widely used in the life sciences and pharmaceutical industries. Both have their strengths and weaknesses, and the decision of which one to use is not always straightforward.
In this article, we will delve into the ongoing debate surrounding SAS and R, examining the pros and cons of each and exploring whether SAS is becoming obsolete in the face of R's growing popularity.
<h3>Table of Contents</h3>
<ul><li><strong><a href="#sas-role">SAS and Its Role</a></strong></li><li><strong><a href="#r-popularity">R and Its Growing Popularity</a></strong></li><li><strong><a href="#shiny-fda">Shiny Apps and FDA Acceptance</a></strong></li><li><strong><a href="#data-standards">Data Standards and Automation in Life Sciences/Pharma</a></strong></li><li><strong><a href="#format-transition">Transition to Modern Data Formats</a></strong></li><li><strong><a href="#key-takeaways">Key Takeaways</a></strong></li><li><strong><a href="#conclusion">Conclusion</a></strong></li></ul>
<h2 id="sas-role">SAS and Its Role</h2>
SAS, or Statistical Analysis System, is a traditional and well-established statistical analysis software that has played a pivotal role in the life sciences and pharmaceutical industries for several decades.
Its historical relevance in these sectors cannot be overstated, as it has been a cornerstone in supporting data analysis, reporting, and decision-making processes.
<h3>Historical Relevance</h3>
<ul><li><strong>Longevity:</strong> SAS has been a trusted companion in the life sciences and pharmaceutical industries since the 1970s. Its long history in these sectors has allowed it to develop a strong foothold and a rich ecosystem of tools and resources tailored to the specific needs of researchers and analysts.</li><li><strong>Regulatory Compliance:</strong> One of the key advantages of SAS is its long-standing reputation for compliance with regulatory requirements, such as those imposed by the U.S. Food and Drug Administration (FDA). This makes it a preferred choice for industries where adherence to stringent regulations is essential.</li></ul>
<h3>Specific Use Cases and Advantages</h3>
<ul><li><strong>Clinical Trials:</strong> SAS is widely used for the analysis of clinical trial data. Its robust capabilities for data management, statistical modeling, and reporting make it a valuable asset in designing and evaluating clinical studies. It allows researchers to perform complex analyses, calculate endpoints, and generate regulatory-compliant reports efficiently.</li><li><strong>Data Integration:</strong> In the life sciences and pharmaceutical sectors, data often come from a variety of sources, including electronic health records, laboratory experiments, and patient data. SAS excels in data integration, allowing users to merge, clean, and prepare data from different sources for analysis seamlessly.</li><li><strong>Customization:</strong> SAS offers a wide range of programming capabilities, allowing users to create customized solutions tailored to their specific needs. This flexibility is particularly valuable in situations where standard analyses do not suffice.</li></ul>
<h3>Challenges and Limitations</h3>
<ul><li><strong>Cost:</strong> One of the primary challenges associated with SAS is its cost. Licensing and maintaining SAS software can be expensive, which can be a barrier for smaller organizations or research projects with limited budgets.</li><li><strong>Learning Curve:</strong> SAS has a steeper learning curve compared to some open-source alternatives like R. This can be a disadvantage for new users or organizations looking to quickly adopt data analysis tools.</li><li><strong>Limited Openness:</strong> SAS is a proprietary software, which means it is less open and extensible compared to open-source alternatives like R. This can limit the ability to integrate it with other tools and libraries.</li></ul>
SAS has a rich history and has been a go-to choice in the life sciences and pharmaceutical industries for a long time. It offers robust features, regulatory compliance, and tailored solutions for specific use cases.
However, its cost, steep learning curve, and limited openness have led some to explore alternatives like R, which we will discuss further in this article.
<h2 id="r-popularity">R and Its Growing Popularity</h2>
R is an open-source programming language and environment for statistical computing and graphics. It was developed by statisticians and data analysts to provide a flexible and powerful platform for data analysis, visualization, and modeling.
Over the years, R has gained substantial popularity in the life sciences and pharmaceutical industries, becoming a prominent choice for data analysis and research.
<h3>The Growing Popularity of R</h3>
<ul><li><strong>Open-Source Nature:</strong> One of the key reasons for R's increasing popularity is its open-source nature. R is freely available, which makes it accessible to a wide range of users, including researchers, analysts, and organizations with varying budgets. This open-access model fosters collaboration and innovation within the scientific community.</li><li><strong>Extensive Package Ecosystem:</strong> R boasts an extensive library of packages, contributed by a global community of developers. These packages cover a wide spectrum of statistical techniques, data visualization tools, and data manipulation functions. In the life sciences and pharmaceutical industries, these packages can be invaluable for addressing domain-specific challenges and conducting specialized analyses.</li><li><strong>Flexibility and Customization:</strong> R's flexibility allows users to tailor their analyses to specific research needs. Researchers in the life sciences and pharma can write custom scripts and functions to implement unique statistical models or data processing workflows. This adaptability is particularly beneficial when dealing with complex and evolving research questions.</li></ul>
<h3>Specific Advantages of Using R</h3>
<ul><li><strong>Reproducibility:</strong> R facilitates reproducible research by allowing users to document their analyses in a script or notebook format. This transparency is crucial in the life sciences and pharmaceutical sectors, where the accuracy and reliability of analyses can have life-altering consequences.</li><li><strong>Data Visualization:</strong> R offers powerful data visualization capabilities through packages like ggplot2. Visualization is essential for understanding complex biological or clinical data, and R's capabilities in this area have contributed to its popularity.</li><li><strong>Integration and Data Interoperability:</strong> R can seamlessly integrate with a variety of data sources, including databases, spreadsheets, and web APIs. This makes it easier for researchers to work with diverse data types commonly encountered in life sciences and pharma research.</li><li><strong>Community Support:</strong> R has a vibrant and active user community, which provides extensive online resources, forums, and user groups. Researchers can readily find support, share knowledge, and collaborate on projects related to their field.</li></ul>
In addition to the technical advantages of using R in the life sciences and pharmaceutical sectors, there are several business advantages to consider:
<ul><li><strong>Cost-Effectiveness:</strong> is an open-source programming language and environment, which means it can significantly reduce software licensing costs compared to proprietary solutions like SAS. This cost-effectiveness is particularly appealing to organizations aiming to optimize their budget allocation.</li><li><strong>Time-to-Insight:</strong> The ability to quickly create data visualizations and conduct analyses in R can expedite decision-making processes in the life sciences and pharmaceutical sectors. This accelerated time-to-insight can be a competitive advantage in a rapidly evolving industry.</li><li><strong>Talent Pool:</strong> With R's popularity and widespread use in academia and research, there is a larger pool of skilled data analysts and statisticians familiar with the language. This makes it easier for organizations to recruit and retain talent in data-driven roles.</li><li><strong>Long-Term Viability:</strong> R has gained substantial traction in the data science and analytics fields, indicating its long-term viability. Choosing a well-established and continuously evolving tool like R can provide confidence in its sustainability for future projects and investments.</li></ul>
R's open-source nature, <strong>rich package ecosystem</strong>, <strong>flexibility</strong>, and <strong>support for reproducibility</strong> have led to its growing popularity in the life sciences and pharmaceutical industries. Researchers and analysts in these fields are increasingly turning to R as a versatile and cost-effective tool for conducting data analysis and advancing their research objectives.
<h3>Challenges and Limitations</h3>
<ul><li><strong>Regulatory Challenges:</strong> While R itself is not a regulatory issue, the use of open-source software like R may raise concerns in regulated environments. Organizations must carefully manage and document their R-based processes to ensure compliance with industry regulations.</li><li><strong>Data Security:</strong> In the context of life sciences and pharmaceutical research, where data integrity is critical, especially in clinical trials, the vigilant protection of sensitive information is paramount. Concerns related to data security may naturally emerge when utilizing open-source software like R. Consequently, organizations must establish robust security measures to safeguard their valuable and confidential data.</li><li><strong>Software Validation:</strong> Validating R packages and scripts for use in regulatory submissions can indeed be a rigorous and resource-intensive process in the life sciences and pharmaceutical sectors. However, there are solutions available.</li></ul>
<blockquote>To address the challenges of software validation in the life sciences and pharmaceutical sectors where validating R packages and scripts for regulatory submissions can be rigorous and resource-intensive, consider exploring this insightful article on <a href="https://appsilon.com/exploring-r-package-validation-in-life-sciences/" target="_blank" rel="noopener">R Package Validation in Life Sciences</a>.</blockquote>
<h2 id="shiny-fda">Shiny Apps and FDA Acceptance</h2>
Shiny apps are interactive web applications built using the Shiny framework in R. They play a crucial role in data visualization and analysis in various industries, including the life sciences and pharmaceutical sectors. Notably, <a href="https://www.r-consortium.org/announcement/2023/10/05/shiny-app-successfully-reviewed-by-fda-cder-staff-pilot-2-announcement-2" target="_blank" rel="noopener noreferrer">the FDA has given the green light to the first publicly submitted Shiny application</a>, marking a significant milestone in regulatory acceptance of this tool.
These apps allow users to create dynamic and user-friendly interfaces for exploring data, conducting analyses, and generating reports. In the context of the life sciences and pharma industry, Shiny apps offer several advantages:
<ul><li><strong>Interactive Data Exploration:</strong> Shiny apps enable researchers and analysts to interact with data in real-time. Users can customize plots, apply filters, and adjust parameters, enhancing their ability to explore complex datasets and gain insights quickly.</li><li><strong>Reproducibility:</strong> Shiny apps help ensure reproducibility by encapsulating data analysis workflows into an interactive interface. This allows for transparent and repeatable analyses, which is crucial for regulatory compliance and peer review in these highly regulated industries.</li><li><strong>Data Visualization:</strong> Shiny's integration with data visualization libraries like ggplot2 and Plotly empowers users to create informative and publication-quality plots and graphs. Visualizations are essential for conveying findings effectively to stakeholders and regulatory bodies.</li><li><strong>Customized Reporting:</strong> Shiny apps can generate customized reports, which can be invaluable for documenting and communicating research findings. These reports can be tailored to meet regulatory requirements and provide clear, well-structured summaries of analyses.</li></ul>
<blockquote>Don't miss this enlightening article on <a href="https://appsilon.com/interactive-clinical-reports-shiny-and-quarto/https://appsilon.com/interactive-clinical-reports-shiny-and-quarto/" target="_blank" rel="noopener">Interactive Clinical Reports with Shiny and Quarto</a> to explore how this innovative approach can transform your data presentations and analysis.</blockquote>
<h3>Rhino and FDA Compliance in Shiny Apps</h3>
<img class="wp-image-22190" src="https://webflow-prod-assets.s3.amazonaws.com/6525256482c9e9a06c7a9d3c%2F65b019513c74b4d4f4cf16c2_rhino.webp" alt="rhino" width="192" height="221" /> Rhino
Now, let's discuss <a href="https://rhinoverse.dev/" target="_blank" rel="noopener">Rhino</a>, a part of the <a href="https://pharmaverse.org/e2eclinical/tlg/" target="_blank" rel="noopener noreferrer">Pharmaverse package repository</a>, to assess its influence on Shiny app development and its contributions towards FDA acceptance and regulatory compliance.
Rhino is a specialized tool designed to enhance Shiny app development in regulated environments, such as the pharmaceutical industry. Here's how using Rhino for Shiny apps can impact FDA acceptance and regulatory compliance:
<ul><li><strong>Validation and Quality Assurance:</strong> Rhino is equipped with features to support validation and quality assurance processes. It allows for the creation of Shiny apps that adhere to industry standards and regulatory guidelines, making it easier to demonstrate compliance with the FDA.</li><li><strong>Audit Trails:</strong> Rhino provides audit trail functionality, which is crucial for tracking user interactions and changes made within a Shiny app. This feature ensures that all data manipulations and analyses are well-documented, helping with auditability and transparency—a critical aspect of FDA acceptance.</li></ul>
Shiny apps are powerful tools for data visualization and analysis in the life sciences and pharmaceutical industries. When used in combination with Rhino, these apps can enhance regulatory compliance and increase the likelihood of FDA acceptance. Rhino's features for validation, audit trails, security, compliance documentation, and regulatory expertise make it a valuable asset in the development of Shiny apps for highly regulated environments.
<blockquote>Interested in how R and Shiny are advancing FDA clinical trial processes? Check out our '<a href="https://appsilon.com/fda-clinical-trial-submissions-with-r-shiny-rhino/" target="_blank" rel="noopener">Advancing FDA Clinical Trial Submissions with R</a>' article for insights.</blockquote>
<h2 id="data-standards">Data Standards and Automation in Life Sciences/Pharma</h2>
Data standards are of paramount importance in the life sciences and pharmaceutical industries. They play a crucial role in ensuring data quality, consistency, and regulatory compliance throughout the drug development process. Here's why data standards are essential:
<ul><li><strong>Regulatory Compliance:</strong> Regulatory agencies, such as the FDA, require standardized data formats to facilitate the review and approval of drug submissions. Adherence to data standards is a regulatory mandate to ensure the integrity and reliability of clinical trial data.</li><li><strong>Data Consistency:</strong> Data standards help maintain consistency across different studies and datasets. When multiple studies are conducted during drug development, standardized data formats enable easy integration and comparison of data, ensuring accurate assessments of safety and efficacy.</li><li><strong>Efficiency and Cost Reduction:</strong> Standardized data collection and reporting processes streamline workflows, reduce errors, and ultimately lower operational costs. This efficiency is especially important in the resource-intensive life sciences and pharmaceutical sectors.</li><li><strong>Interoperability:</strong> Data standards enable interoperability between different software systems and platforms. This ensures that data can be exchanged and analyzed seamlessly, promoting collaboration among research teams, regulatory agencies, and industry stakeholders.</li></ul>
<h3>CDISC Standards and the Oak Package</h3>
<img class=" wp-image-22193" src="https://webflow-prod-assets.s3.amazonaws.com/6525256482c9e9a06c7a9d3c%2F65b01951725ded127af09ccd_cdisc.webp" alt="cdisc" width="313" height="164" /> cdisc
The <a href="https://www.cdisc.org/" target="_blank" rel="noopener noreferrer">Clinical Data Interchange Standards Consortium (CDISC)</a> is a global nonprofit organization that develops and maintains standards for clinical and nonclinical research data. CDISC standards are widely adopted in the pharmaceutical industry. One important CDISC standard is the <a href="https://pharmaverse.org/e2eclinical/sdtm/" target="_blank" rel="noopener noreferrer">Study Data Tabulation Model (SDTM)</a>, which provides guidelines for the structure and content of analysis datasets used for regulatory submissions.
<a href="https://www.cdisc.org/oak" target="_blank" rel="noopener noreferrer">Oak</a> is an open-source project designed to automate the creation of SDTM (Study Data Tabulation Model) tables in compliance with CDISC standards. It streamlines the transformation of raw clinical trial data into analysis-ready datasets, reducing manual effort and the risk of errors. Oak helps ensure that ADaM datasets are generated consistently and in accordance with CDISC standards, thus facilitating regulatory compliance.
<h3>The Admiral Package in the Pharmaverse Repository</h3>
<img class=" wp-image-22195" src="https://webflow-prod-assets.s3.amazonaws.com/6525256482c9e9a06c7a9d3c%2F65b019537f2730eb54c0a0ab_admiral.webp" alt="" width="197" height="228" /> admiral
<a href="https://cran.r-project.org/web/packages/admiral/index.html" target="_blank" rel="noopener noreferrer">Admiral</a> is another R package within the Pharmaverse repository, primarily focusing on the development of ADaM datasets for use in pharmaceutical industry applications, especially in clinical trials. It significantly aids in the structured creation and management of these datasets, which are foundational for summarizing key study results such as efficacy and safety data.
Utilizing the Admiral package streamlines the process of dataset development, indirectly supporting the generation of tables and reports. This ensures that outputs are consistent and comply with industry standards, which is critical in regulatory submissions and research reporting.
<h2 id="format-transition">Transition to Modern Data Formats</h2>
The shift from .xpt files to dataset JSON format represents a transition towards more modern and flexible data formats in the realm of data handling and analysis. Let's explore this transition and its impact on these processes:
<h3>.xpt Files (SAS Transport Files):</h3>
Historically, .xpt files, also known as SAS Transport Files, were commonly used for storing and exchanging clinical and research data in the pharmaceutical and life sciences industries. .xpt files were a proprietary binary format associated with SAS (Statistical Analysis System), a widely used software for data analysis.
While .xpt files served their purpose, they had limitations in terms of interoperability and transparency. They were primarily designed for use within the SAS software ecosystem.
<h3>Dataset JSON Format</h3>
<p style="text-align: left;"><a href="https://www.cdisc.org/dataset-json" target="_blank" rel="noopener noreferrer">Dataset-JSON</a> (JavaScript Object Notation) has become increasingly relevant in data handling and analysis, notably in industries like life sciences and pharmaceuticals. JSON's modern and versatile nature, coupled with its human-readable, lightweight, and platform-independent format, aligns well with the data processing capabilities often employed by R users in these sectors.</p>
In the context of data handling and analysis for the life sciences, <a href="https://www.clinicalleader.com/doc/no-more-xpt-piloting-new-dataset-json-for-fda-submissions-0001" target="_blank" rel="noopener noreferrer">dataset-JSON</a> format provides several advantages:
<ul><li><strong>Interoperability:</strong> The broad adoption of JSON across programming languages (including R), data analysis tools, and web applications underscores its versatility. This flexibility facilitates the seamless exchange and manipulation of data across various software environments, making it a valuable asset for researchers and analysts in these domains.</li><li><strong>Transparency:</strong> JSON files are human-readable, making it easier to understand the data's structure and contents, which is valuable for data validation and troubleshooting.</li><li><strong>Flexibility:</strong> JSON allows for nested and hierarchical data structures, making it suitable for representing complex datasets with multiple levels of information.</li><li><strong>Version Control:</strong> JSON files can be efficiently managed and version-controlled using tools like Git, enhancing data traceability and reproducibility.</li></ul>
<h4>Impact on Data Handling and Analysis</h4>
<ul><li><strong>Enhanced Compatibility:</strong> The transition to dataset JSON format improves compatibility with a broader set of data analysis tools and platforms. Researchers and analysts can leverage a wider range of software options for their data analysis needs.</li><li><strong>Data Transparency:</strong> JSON's human-readable format enhances data transparency, making it easier to review, validate, and understand datasets. This is particularly important in highly regulated industries like pharmaceuticals, where data integrity is crucial.</li><li><strong>Efficient Data Exchange:</strong> JSON simplifies data exchange between collaborators, research teams, and organizations, as it doesn't rely on proprietary formats or software dependencies.</li><li><strong>Flexibility and Scalability:</strong> JSON's flexibility allows it to accommodate evolving data structures and requirements, making it suitable for both small-scale studies and large, complex datasets.</li></ul>
The transition from .xpt files to dataset JSON format represents a positive shift towards more modern and versatile data formats, particularly in the context of R within the life sciences and pharmaceutical industries. This transition improves data handling, enhances data analysis capabilities, and promotes greater interoperability and transparency, ultimately contributing to more efficient and effective research and development processes.
<h2 id="key-takeaways">Key Takeaways</h2><ul><li><strong>Significance of Data Analysis:</strong> Data analysis and handling are of paramount importance in the life sciences and pharmaceutical sectors, influencing critical decisions in drug development, clinical trials, and regulatory compliance.</li><li><strong>SAS's Historical Relevance:</strong> SAS, a traditional statistical analysis software, has a longstanding history and reputation in these industries. It offers advantages such as regulatory compliance and specialized tools for clinical trials and data integration.</li><li><strong>R's Growing Popularity:</strong> R, an open-source programming language and environment, is gaining popularity due to its flexibility, extensive package ecosystem, and cost-effectiveness. It empowers researchers with customization, data visualization, and transparency benefits.</li><li><strong>Shiny Apps and Regulatory Compliance:</strong> Shiny apps, created using R, are instrumental in data visualization, analysis, and regulatory compliance. Tools like Rhino enhance Shiny app development, ensuring adherence to regulatory standards.</li><li><strong>Data Standards and Automation:</strong> Adherence to data standards, such as CDISC, is crucial for regulatory compliance. Automation tools like the Oak and Admiral packages streamline data transformation and table generation, improving efficiency and accuracy.</li><li><strong>Transition to Modern Data Formats:</strong> The shift from .xpt files to dataset JSON format signifies a move toward modern, interoperable, and transparent data formats that enhance data handling and analysis in pharmaceutical and life sciences research.</li></ul>
<h2 id="conclusion">Conclusion</h2>
In this article, we explored the critical considerations surrounding the choice between SAS and R in the life sciences and pharmaceutical industries.
In the debate between SAS and R, there is no one-size-fits-all answer. Both have their strengths and limitations, and the choice should be driven by the specific needs and regulatory requirements of the project or organization.
Are you looking to move from SAS to R seamlessly? We are the right partners for this transition; <a href="https://appsilon.com/#contact" target="_blank" rel="noopener">let’s make it happen together</a>.