Data for Good: Our approach to tackling climate change with data science
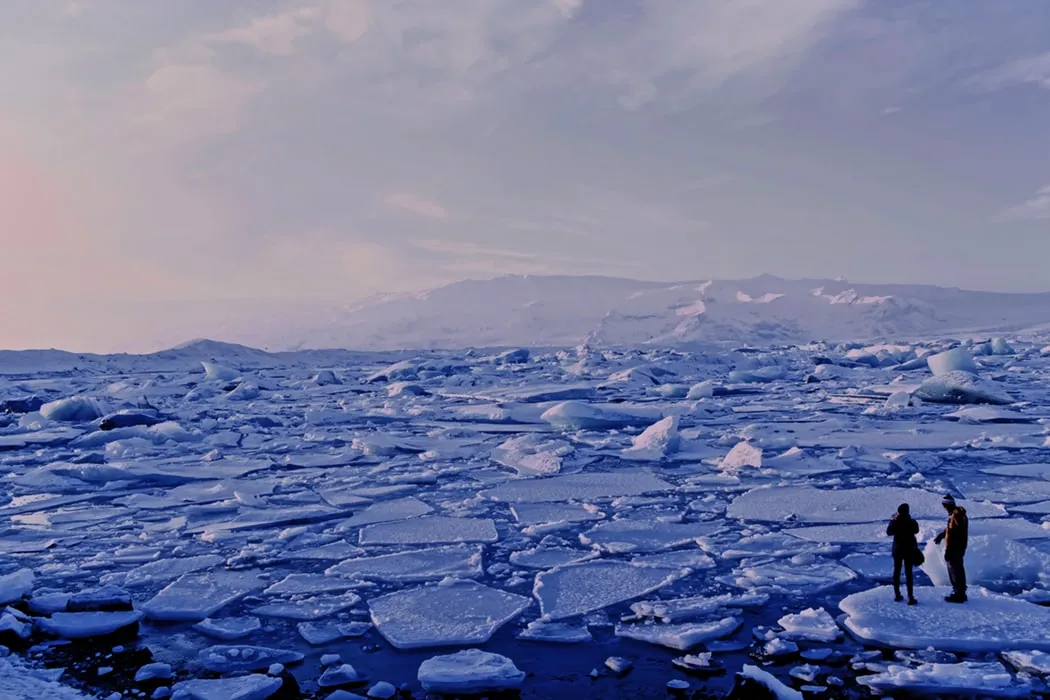
Technology has been a tool that has brought unprecedented prosperity to humanity. It allowed us to extract resources, transform, and transport them with ever-increasing efficiency. It brought about the contemporary global economy, <a href="https://esajournals.onlinelibrary.wiley.com/doi/10.1890/070062" target="_blank" rel="noopener noreferrer">transforming 75% of all land in the process</a>. It has spawned innovation and created paths to track a wealth of data. However, this prosperity has come at a cost to our planet and its ecosystems to an extent, which now poses an existential threat to us and all other species on earth. This technology and its ensuing progress, are beginning to show their influence on the world, but can our technology and data be used for good? Indeed, in spite of what some prominent public figures might claim, it has recently been revealed that far from being “alarmists”, <a href="https://blogs.scientificamerican.com/observations/scientists-have-been-underestimating-the-pace-of-climate-change/" target="_blank" rel="noopener noreferrer">the scientific community has in fact been underestimating the unfolding of climate change and its impacts</a>. We might be headed towards reaching the widely quoted figure of global 2°C increase much faster than anticipated, by which point feedback loops in ecosystems might make any mitigation efforts fruitless. We are already getting a taste of what is likely to come in the coming decades as our planet warms – droughts, fires, flooding, storms, <a href="https://climate.nasa.gov/effects/" target="_blank" rel="noopener noreferrer">all growing in severity</a>. The associated social and biodiversity issues emerge with increasing climate-related migration of people from the most affected areas and the sixth mass extinction of plants and animals, now dubbed the <a href="https://link.springer.com/article/10.1007/s12210-013-0258-9" target="_blank" rel="noopener noreferrer"><b>Anthropocene</b> extinction</a>, for having its roots in human activity. The international community, a network of concerned governmental actors, academia, NGOs, social businesses, and activists are addressing climate change through mitigation and adaptation efforts. <b>Mitigation</b> entails reducing greenhouse gas emissions through innovation in electricity systems, industry, agriculture, forestry, and cities. <b>Adaptation</b> recognizes that regardless of our mitigation efforts, changes to the climate are inevitable and employs solutions to better predict the emerging risks to plan for resilience and disaster management. Technology is being applied to assist in these efforts. <b>Machine Learning</b> <b>(ML)</b> and <b>Artificial Intelligence (AI)</b>, being at the forefront of technological innovation, are yet to be fully utilized. The rate of progress in this area is exponential and even the practitioners cannot anticipate what the coming decades will bring. Indeed, some problems that had been considered virtually insurmountable 5 or 10 years ago have now been solved. Without a doubt, ML and AI are powerful tools that can be used to fight the greatest threats facing our planet. However, the untapped potential of <b>ML</b> to enhance these efforts requires communication. On the one hand, we have an eager community of ML practitioners, on the other, we have a multitude of stakeholders fighting climate change. Whilst the ML practitioners may find it difficult to find the most impactful areas for their solutions, the stakeholders in the climate change community may lack the resources to identify the ML solutions that might best assist in forwarding their respective causes. <h2>Tackling climate change with Machine Learning and its challenges</h2> <a href="https://arxiv.org/abs/1906.05433" target="_blank" rel="noopener noreferrer">Tackling Climate Change with Machine Learning</a>, a recent paper produced as a collaboration of 22 scholars and practitioners from academia, NGOs, and the private sector, attempts to address this issue. It presents a comprehensive overview of the applications of ML to various areas of the fight against climate change. The work is a valuable resource for both ML practitioners and stakeholders and can serve as an inspiration for what is possible. The authors organize the paper into 13 chapters, each dealing with a different domain of climate change solutions: <img class="alignnone wp-image-14305" src="https://webflow-prod-assets.s3.amazonaws.com/6525256482c9e9a06c7a9d3c%2F65b022c6350258d48b3c4e9a_ML-vs-Climate-Change-blog-post-Google-Docs.webp" alt="domains of climate change solutions" width="600" height="379" /> <img class="alignnone wp-image-14311" src="https://webflow-prod-assets.s3.amazonaws.com/6525256482c9e9a06c7a9d3c%2F65b022c8536cc909ce52651e_domains-of-climate-change-solutions.webp" alt="domains of climate change solutions2" width="600" height="592" /> The relevant areas of ML are then discussed for each of these domains including: <img class="aligncenter wp-image-2718" src="https://webflow-prod-assets.s3.amazonaws.com/6525256482c9e9a06c7a9d3c%2F65b022c85b956c19cd8f1a32_ML-vs-Climate-Change-blog-post-Google-Docs-9-23-2019-11-54-18-AM.webp" alt="domains of machine learning research" width="500" height="521" /> Whilst these lists might not be exhaustive, they provide a starting point for both the IT industry and the climate change stakeholders. Delving deeper into the paper helps to further understand both the challenges in the climate change effort and the different ML solutions available. The paper has intuitive appeal as the authors further classify each particular area’s likelihood of benefiting from ML solutions. While the potential becomes clear, however, it is not without its caveats. The authors draw attention to one such issue, namely the Jevons paradox. The Jevons paradox occurs when the improvement in efficiency generates greater demand for service thereby nullifying or even worsening the anticipated positive outcome. For instance, ML can find applications in precision agriculture. However, this increased precision, whilst hopefully reducing the GHG impact of any particular farming enterprise might in fact lead to greater overall uptake of intensive agriculture in favor of less intensive, sustainable modes of farming. Similar issues have plagued the adoption of new technology to sustainability efforts in general. Recent history is filled with examples of solutions being applied to problems without a <b>holistic approach</b>, which would have allowed us to anticipate undesirable outcomes. For instance, biofuel production became popular a decade ago, when Western countries mandated the use of vegetable oils in biofuels. Fast forward to today, the push towards biofuels resulted in massive deforestation in Indonesia as rainforests were transformed into palm oil farms. Perhaps a less techno-centric approach would have prevented these problems in the first place. <h2>Our approach</h2> Therefore, at Appsilon Data Science we advocate an <b>exploratory approach</b> towards utilizing ML to address climate change. Tech companies can provide inspiration to stakeholders fighting climate change by showcasing the technologies, but the problems should be defined by the practitioners in this area in a collaborative manner. Rather than finding exciting applications for ML solutions, the tech community should welcome inquiries from governments, NGOs, and other actors. To this end, we are launching our <a href="https://appsilon.com/data-for-good/" target="_blank" rel="noopener noreferrer"><b>Data for Good</b></a> initiative. We will be publishing blog posts such as this one to help the development and climate change communities get acquainted with the potential in data science. In the coming weeks, we will also be launching a newsletter showcasing the most exciting ideas and projects already taking place. We encourage stakeholders to <b>share their challenges with us</b>. We are hoping to learn, inspire and work together.
Contact us!
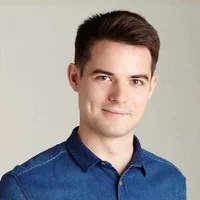