Protecting Gabon Wildlife - Using AI for Biodiversity Conservation

<h2>Table of Contents</h2><ul><li><a href="#anchor-1" rel="noopener noreferrer">Gabon wildlife conservation</a></li><li><a href="#anchor-2" rel="noopener noreferrer">Camera traps & AI</a></li><li><a href="#anchor-3" rel="noopener noreferrer">Mbaza AI achievements</a></li><li><a href="#anchor-4" rel="noopener noreferrer">Next steps</a></li></ul>
The COP26 summit has come to an end after two weeks in Glasgow, Scotland. Although negotiations have been hard-fought, many developing countries leave with nothing more than promises from the top contributors of climate emissions.
If one thing is clear, it’s that nations are failing to reign in greenhouse gas emissions. The <a href="https://unfccc.int/news/updated-ndc-synthesis-report-worrying-trends-confirmed" target="_blank" rel="noopener noreferrer">IPCC reports</a> that emissions must be reduced by 45% within this decade to prevent an increase of global temperatures above 1.5C. And yet, emissions are anticipated to grow 16% by 2030.
<h3>Why strive for 1.5?</h3>
With a global temperature increase of 1.5C, the world is projected to see <a href="https://www.science.org/doi/abs/10.1126/science.289.5487.2068" target="_blank" rel="noopener noreferrer">extremes more frequently</a>. From sweltering heat and droughts to rising sea levels and increased flooding. It’s an ambitious goal, but if we can implement much-needed changes in-line with the 1.5C limits, we might be able to mitigate these effects. If we fail (and it looks like we will) then more drastic measures will be required.
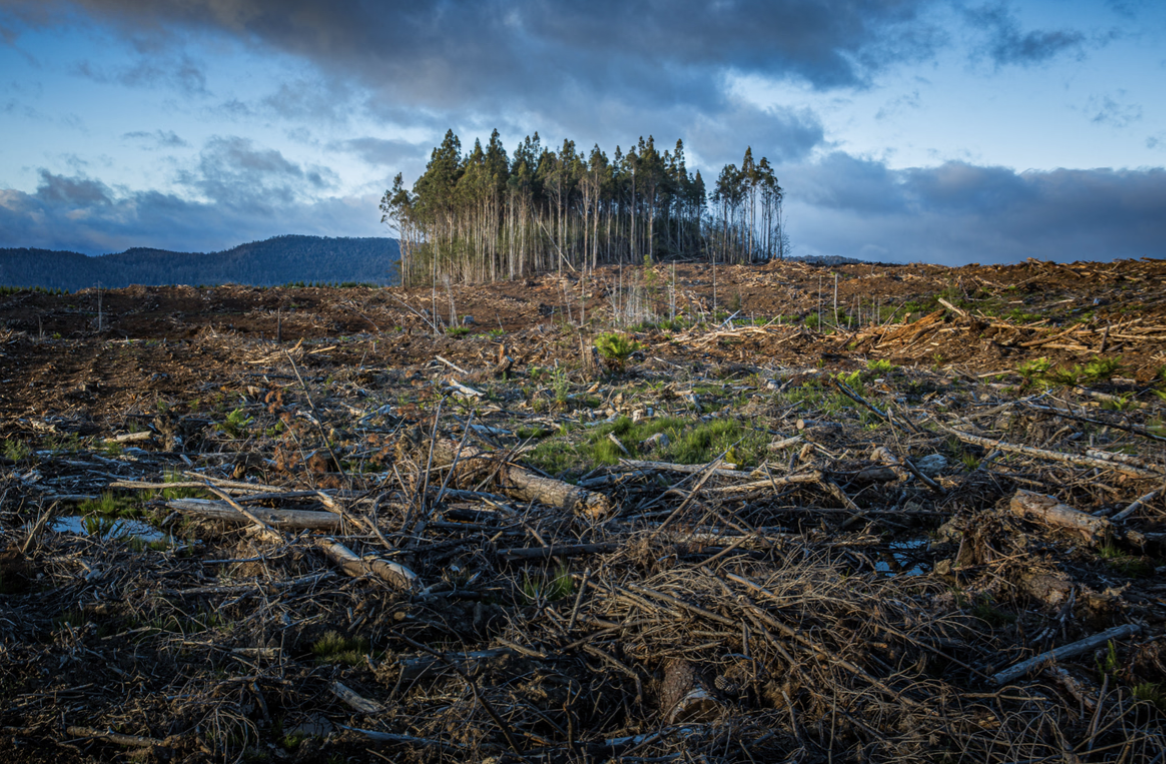
The resulting decline in biodiversity will have far-reaching effects beyond global fisheries and vanishing coral reefs. There will be a rise in poverty, particularly in at-risk populations most vulnerable to the effects of climate change. The changing climate will adversely affect crops and livestock, disrupting global food supply chains. And health risks will rise with increased heat-related deaths and a new dawn of vector-borne diseases.
<h3>A way forward</h3>
But in a world with such a bleak future, individuals and organizations are finding and implementing real, tangible solutions. As Appsilon has grown, we’ve come to realize our experience as data science professionals can play an important role in the fight against climate change. That’s why, through our Data for Good initiative, we apply our expertise to climate change and environmental protection projects.
Our efforts to assist biodiversity conservation practitioners and researchers have recently led to the development and adoption of AI-enabled camera traps to remotely monitor wildlife. In this particular case study, automated camera traps have been deployed in the Gabon tropical forest. The result is an opportunity to monitor biodiversity in real-time, improve wildlife resource management, promote wildlife tourism, and detect illegal activities at scale.
Continue reading to learn how Appsilon assisted researchers from the University of Stirling in their collaboration with Panthera Corp. and the National Parks Agency in Gabon to improve Gabon wildlife conservation.
<h2 id="anchor-1">Gabon wildlife conservation</h2>
The Congo Basin is a source of extraordinary biodiversity, but that biodiversity is under a very real threat. As the second-largest area of rainforest in the world, the Congo Basin houses roughly 400 species of mammals and 1,000 species of birds. Among those are endangered wildlife like forest elephants, bonobos, lowland gorillas, and chimpanzees. Fortunately, Gabon, the African nation with the largest area of forest preserve, is betting big on its rainforest and people are beginning to take notice.
<img class="size-full wp-image-3665" src="https://webflow-prod-assets.s3.amazonaws.com/6525256482c9e9a06c7a9d3c%2F65b01cbd832c47d673110b71_chimpanzee-mother-and-infant.webp" alt="Chimpanzee mother and infant in Lopé National Park, Gabon" width="1600" height="900" /> Chimpanzee mother and infant in Lopé National Park, Gabon (Image courtesy of ANPN/Panthera)
Gabon recently received $17 million from Norway to protect its forests. This comes from a nearly $150 million pledge from Norway as part of the Central African Forest Initiative (CAFI). But exactly how Gabon will protect its forest, an important carbon sink and biodiversity hotspot, while maintaining a healthy economy is a bit of an unknown.
<h3>Sustainable logging</h3>
As the oil industry in the region begins to wane, Gabon Forests Minister Lee White is looking to sustainable logging as part of a greener economy. The Forests Minister is promoting tighter regulation and focuses on the local processing of materials to encourage better forest management and job creation. The African Development Bank has projected Gabon could add nearly $1.5 billion to its economy by developing its local timber production.
However, sustainable logging is a tight rope to balance. And to ensure sustainability, Gabon is stiffening up regulations by requiring industry certifications as well as implementing timber-tracing systems and monitoring forest cover via satellite imagery.
<blockquote><strong>Gain a better vantage with your R project by applying <a href="https://appsilon.com/deep-learning-in-satellite-imagery/" target="_blank" rel="noopener noreferrer">Deep Learning for satellite imagery</a>.</strong></blockquote>
Among the concerns for this so-called Green Gabon movement, is the preservation of Gabon’s wildlife. As Gabon begins to crack down on illegal logging, it has an opportunity to incorporate state-of-the-art monitoring systems and conservation methods.
<h2 id="anchor-2">Camera Traps & Artificial Intelligence</h2>
Threats from unmanaged logging, poaching, and other illegal activities can be challenging to monitor across such a large and wild terrain as the Congo Basin. And ultimately these detrimental activities may go undetected for years. Artificial Intelligence (AI) and remotely sensed data in the form of camera traps provide an opportunity to tackle these issues, but until recently were limited by technical barriers.
The main limitations are transmitting the camera trap imagery for real-time analysis without reliable satellite internet and the long delays caused by manual data analysis.
<img class="aligncenter size-full wp-image-8830" src="https://webflow-prod-assets.s3.amazonaws.com/6525256482c9e9a06c7a9d3c%2F65b01de040d120c4e25661f3_manual-identification-process.webp" alt="Simplified workflow for manual identification of camera trap imagery" width="891" height="432" />
And so with the help of Appsilon's ML team, researchers at the University of Stirling successfully implemented one such solution tackling the latter issue - the Mbaza AI.
It is worth noting that there is also <a href="https://www.biorxiv.org/content/10.1101/2021.11.10.468078v1?rss=1" target="_blank" rel="noopener noreferrer">promising research concerning the former</a> limitation, in which Appsilon's ML team was also involved, providing the ML models.
<h3>Mbaza AI project background</h3>
In 2020, Appsilon was given the opportunity to contribute to biodiversity conservation efforts at the National Parks Agency in Gabon. This work was in collaboration with researchers from the University of Stirling with support from the Panthera Corp and funding from the Google for Education fund.
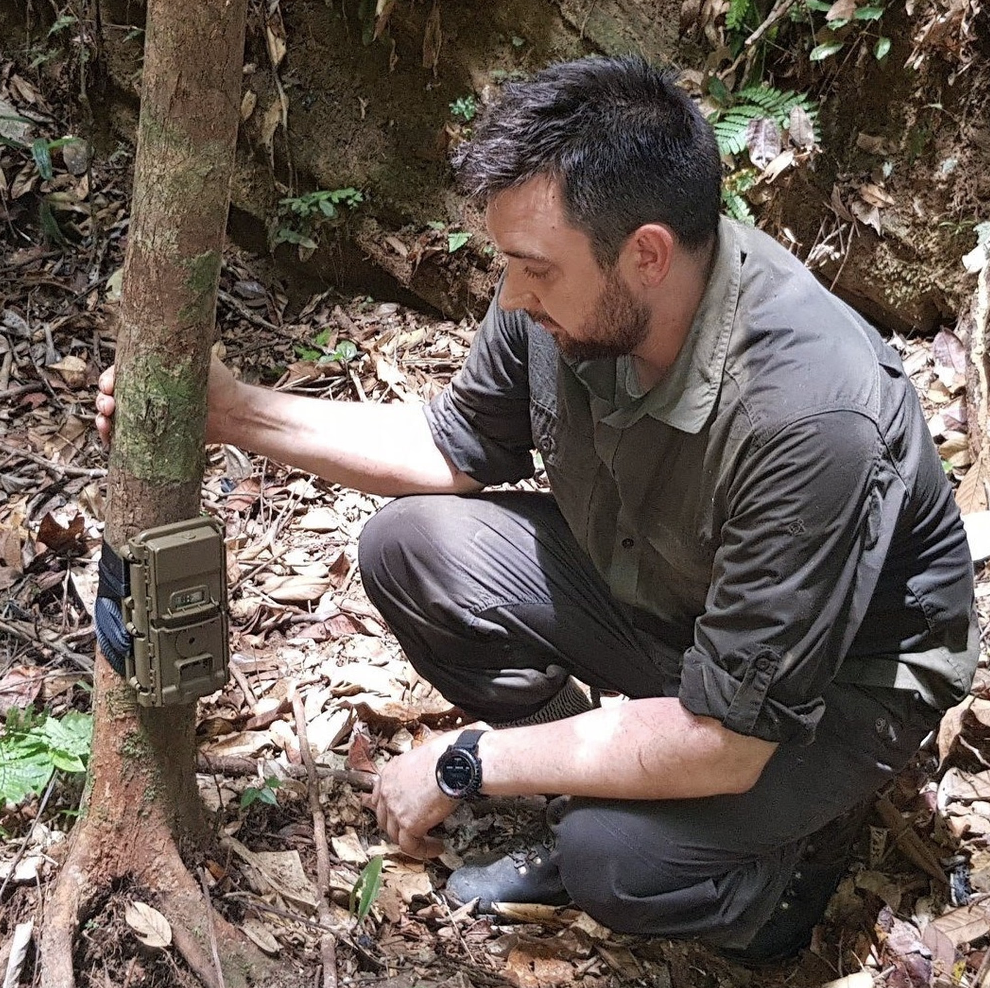
The goal was to resolve the aforementioned barriers and create an easy-to-use, open-source, and scalable software tool called Mbaza AI. Mbaza AI serves to detect and classify wildlife automatically from camera trap images using computer vision. The camera traps and their built-in ML models can be deployed across a wide range of environments. Requiring only a small training dataset of the regional wildlife. The application also needed to be standalone, available offline to be a practical solution for large-scale adoption. Use and management of the tool should also require no prior experience with programming.
<img class="aligncenter wp-image-8831 size-full" src="https://webflow-prod-assets.s3.amazonaws.com/6525256482c9e9a06c7a9d3c%2F65b01fb4ccf2ef0d95c4eb2e_model-identification-process.webp" alt="Simplified workflow for automated, ML model identification of camera trap imagery" width="874" height="428" />
<h2 id="anchor-3">Mbaza AI achievements</h2>
Applying machine learning algorithms to improve camera trap data processing is not a new concept. What differentiates the task we set out to accomplish is the ability to run a high-performance ML model capable of fully automated image labeling. Cutting out the time-intensive and often imperfect manual identification of species.
<img class="size-full wp-image-8873" src="https://webflow-prod-assets.s3.amazonaws.com/6525256482c9e9a06c7a9d3c%2F65b01fb66c7e8f92760ae6cc_elusive-Nkulengu-Rail-1.webp" alt="Camera trap image of an elusive bird, Nkulengu Rail" width="640" height="442" /> Can you spot the elusive Nkulengu Rail? Hint: it's a bird with orange legs. (Image courtesy of ANPN/Panthera)
The problem with most ML models is that accuracy levels are too low to be fully relied upon. Sure, an imprecise or inaccurate model can be used to speed up the identification pipeline. But at some point, human eyes will need to manually scan and verify those images. The result is further delays and missed opportunities. It's a bottleneck that unnecessarily limits researchers, land managers, and other stakeholders.
And so Stirling University researcher Robin Whytock, Ph.D., Appsilon ML Lead Jędrzej Świeżewski, Ph.D., and a team of researchers developed, tested, and successfully applied an ML model to achieve a fully automated analysis of camera trap data. <a href="https://besjournals.onlinelibrary.wiley.com/doi/10.1111/2041-210X.13576" target="_blank" rel="noopener noreferrer">The case study they used</a> classified Central African forest mammalian and avian species. And even with a relatively small dataset of 300,000 images used to train the model, the outcome was strong.
<img class="size-full wp-image-8874" src="https://webflow-prod-assets.s3.amazonaws.com/6525256482c9e9a06c7a9d3c%2F65b01cba952f213de6d1f07f_ML-model-spots-elusive-bird-1.webp" alt="Camera trap image with blue outline on ML identified bird" width="640" height="442" /> Mbaza AI model can pick out subtle identifiers including the orange leg of the Nkulengu Rail.
<h3>Mbaza AI performance</h3>
We measured Model performance based on three ecological metrics:
<ol><li>Species richness - to quantify species count both temporally and spatially</li><li>Activity patterns - to determine activity and life behavior traits</li><li>Occupancy - a hierarchical model to account for imperfect detection</li></ol>
The metrics selected ensured the model satisfied the real needs of researchers in an ecological context. Ultimately, we wanted to avoid a techno-centric solution that did not satisfy the end-users. And with close collaboration with world-class experts on biodiversity conservation, we were able to carefully navigate and avoid this.
The outcome of the ML algorithm was a 90% accuracy for predictions on out-of-sample data (for many of the species). The model can classify roughly 4,000 images per hour on desktop machines used by park rangers and ecologists in the field, without access to powerful cloud computing resources. It reduces the time needed to analyze thousands of trap images from several weeks to a single day. The results of the case study also indicate that although more training data is nice to have, it’s not necessary to accurately and precisely label images using an ML model. In all, Mbaza AI successfully showcases full automation of camera trap image analysis is possible. And in being open-source, researchers across the globe can freely access the tool. Improving the opportunity for large-scale adoption.
<h2 id="anchor-4">Next steps - moving beyond Gabon wildlife</h2>
The Mbaza AI project helped resolve the limitations of manual identification. But this still leaves behind the issue of transmitting the images for real-time data analysis in remote areas.
Recently, Robin Whytock and researchers from the Netherlands (Thijs Suijten and Tim van Deursen from Hack the Planet, Q42) successfully implemented a modified camera setup that solves this missing link. It uses a separate model provided by Appsilon's ML team. The AI instantly labels an image and sends an ‘alert’ message with the label and metadata via a satellite network. <a href="https://www.biorxiv.org/content/10.1101/2021.11.10.468078v1.full.pdf+html" target="_blank" rel="noopener noreferrer">The pilot test</a> has proven successful and results show the system can remain operational for up to three months without the need for boots on the ground. Furthermore, the end-user can receive an alert within minutes allowing for rapid responses.
With further technological advances, the potential for AI and remotely sensed data applications could prove immensely beneficial. The ability to improve real-time monitoring, resource management, and detection of illegal activity - at scale - is one instance data science can be used to mitigate the impacts of climate change.
<h3>Let's make an impact together</h3>
As the world moves forward from COP26, with unsatisfactory agreements, individuals and organizations need to push for action. Not just by voicing concerns and demands, but by creating and implementing solutions. We are more committed than ever to supporting researchers and organizations building real solutions. We are open to cooperation and actively looking for ways to apply our data science expertise to climate change projects.
If you are an NGO, academic institution, or public benefit corporation we encourage you to reach out to us with your social good projects. The Appsilon team has developed unique solutions for Fortune 500 companies, NGOs, and nonprofits for years. We have significant expertise in interactive data visualization and machine learning (in particular computer vision). And we are open to collaborating on projects that align with our Data for Good mission.
The time for discussion is waning. Now is the time for solutions. We can help you by leveraging our skillset to have a positive impact on our planet. Turning software development into powerful insight. Reach out to us [<a href="mailto:hello@wordpress.appsilon.com">hello@wordpress.appsilon.com</a>] and let’s turn ideas into action.