Decision Making Support Systems #3: Differences between IA and AI
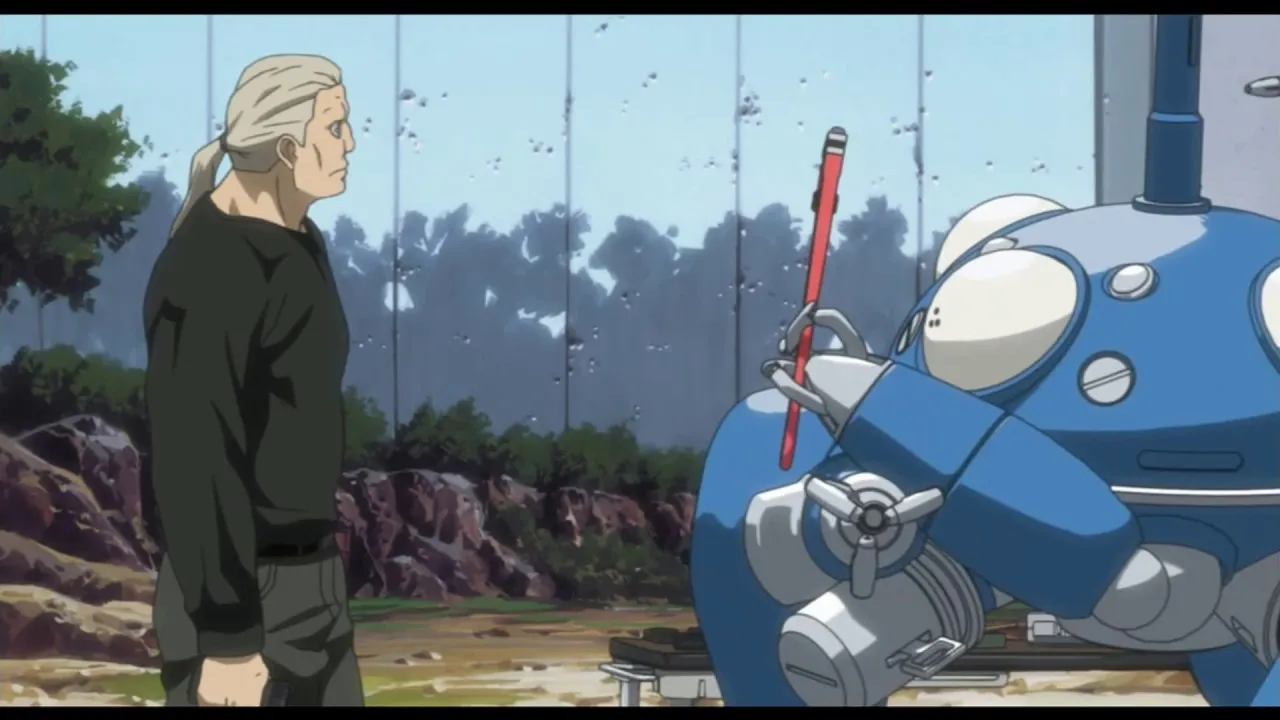
<h2>The Differences between Artificial Intelligence and Augmented Intelligence</h2> In previous posts, we looked at the definition of Artificial Intelligence (AI) and the definition of Intelligence Augmentation (IA). So, what are the differences between the two? Intelligence Augmentation has always been concerned with aiding human decision making and keeping humans-in-the-loop, whereas the AI endeavor seeks to “<a href="https://www.stateof.ai/">build machines that emulate and then exceed the full range of human cognition</a>, with an eye on replacing them. Rob Bromage puts it well in “<a href="https://www.hcamag.com/au/news/general/artificial-intelligence-vs-intelligence-augmentation/152265">Artificial intelligence vs intelligence augmentation</a>”: “AI is an autonomous system that can be taught to imitate and replace human cognitive functions. To put it simply, the machine completely replaces human intervention and interaction. <b>IA</b>, on the other hand, plays more of an assistive role by leveraging AI to <b>enhance</b> human intelligence, rather than replace it.” In “<a href="https://medium.com/datadriveninvestor/augmented-intelligence-not-artificial-intelligence-is-the-future-f07ada7d4815">Augmented Intelligence, not Artificial Intelligence, is the Future</a>” Aaron Masih writes that “While the underlying technologies powering AI and IA <b>are the same</b>, the goals and applications are fundamentally different: AI aims to create systems that run without humans, whereas IA aims to create systems that make <b>humans</b> better.” <img class="wp-image-3023 size-full" src="https://webflow-prod-assets.s3.amazonaws.com/6525256482c9e9a06c7a9d3c%2F65b022bc2e8e917bd49f9d65_IlluminatiWatcherDotCom-TARS-CASE-Monolith.webp" alt="A still from the film "Interstellar." Astronaut and robot working together" width="820" height="420" /> A beneficial partnership between human and machine is depicted in <a href="https://slate.com/technology/2014/11/tars-the-interstellar-robot-should-be-the-future-of-artificial-intelligence.html">Interstellar</a>. IA systems are able to exceed system boundaries (the parts of an environment not covered by an AI model's data inputs) because of the “unsung heroes of the AI revolution” -- humans! AI systems can only work with the datasets that they’re trained on and that feed them. Subject matter expert humans excel in applying context and intuition to problems. Humans can grasp and explain <strong><a href="http://bayes.cs.ucla.edu/WHY/">causality</a></strong>. Alex Bates recalls from his experience running a an AI/prescriptive maintenance firm that “...what was remarkable about the human process engineers and maintenance engineers at these plants ...were all the clues they incorporated into their assessments of equipment failure, allowing them to identify what was failing and what to repair. Where they struggled, and where the AI helped, was in making sense of all the massive amount of sensor data coming off the equipment.” Another major difference? To state the obvious, one receives much more attention than the other. Even the casual industry observer of the industry that <b>augmenting human intelligence</b> represents a tiny fraction of the total research and development devoted to Artificial Intelligence. The lack of investment in the area seems like a missed opportunity. So the AI approach seeks to minimize or replace the role of humans, while an IA solution seeks to amplify the abilities and performance of the humans that participate in a given activity. The IA approach benefits from the human ability to think outside system boundaries. But despite the lack of investment and press attention on Intelligence Augmentation, businesses and other organizations continue to benefit from IA applications... <h2>Examples of IA at work today </h2> <img class="wp-image-3026 size-full" src="https://webflow-prod-assets.s3.amazonaws.com/6525256482c9e9a06c7a9d3c%2F65b271beab6dc21064ed51f4_filip.webp" alt="Appsilon CEO Filip Stachura presenting "Why Shiny" at useR Toulouse 2019 " width="480" height="360" /> CEO of Appsilon Filip Stachura at <a href="http://CEO of Appsilon Filip Stachura at useR! Toulouse 2019">useR! Toulouse 2019</a> I asked Filip Stachura, CEO of Appsilon Data Science, who are specialists in Data Science and Machine Learning, <i>How much of what you do as a company qualifies as Intelligence Augmentation</i>? <b>FS:</b> Most of what we do qualifies as IA. <b>Decision support systems</b> can be more realistic in many business cases. Even a recommendation engine application is a sales-support decision system, not the AI that sells itself. FS: For one firm we did the following: Prices for products that our client sells change frequently and depend heavily on negotiations. When a client requests a product, the salesperson opens the application that we built and fills in the client and product name. They then see the history of deals made with the client and <b>suggested</b> prices/discounts for the product. The prices vary depending on the segment of the business, category/size of the client and the size of the order. Ultimately, the human salespeople make the pricing decision, but the application provides the most up to date information to optimize decision-making. It’s sales-support, not salesperson replacement. FS: Here is another example. What if a manager has to optimize the usage of hundreds of varying models of cleaning machines that are based in different locations in a region? Each location has its own logistical needs and have staff of varying levels of expertise in operating the machines. How do you optimize performance and cost with such diverse conditions? Is the answer to eliminate the manager in charge, or is it to augment the manager’s capabilities with a decision-support system? Obviously it’s the latter, since a fully automated management system would have great difficulty in assessing non-standard situations and communicating with the various teams at the various locations. Appsilon Data Science co-founder <a href="https://twitter.com/D_Rodziewicz">Damian Rodziewicz</a> added that <strong>DR</strong>: We also worked with <a href="https://twitter.com/kenbenoit">Dr. Ken Benoit</a> and his team from the London School of Economics to make their <a href="https://quanteda.io/"><strong>Quanteda</strong> text analysis tool</a> available to a much greater number of social scientists and practitioners from other fields, including medicine and law. Now the user doesn't need to know the R programming language in order to make use of the powerful Quanteda R package. A human researcher can use the Quanteda package to quickly evaluate language and social trends from millions of documents, truly extending the human's research domain by many orders of magnitude. <img class="wp-image-3024 size-large" src="https://wordpress.appsilon.com/wp-content/uploads/2019/10/kenquanteda-1024x768.jpeg" alt="Dr. Ken Benoit of the Quanteda projeect" width="1024" height="768" /> The man and the machine: Dr. Ken Benoit and the <a href="https://quanteda.io/index.html">Quanteda</a> text analysis tool Here is another example, and I have transcribed and hijacked it from the Andrew Ng “<a href="https://www.deeplearning.ai/blog/andrew-ng-at-amazon-remars-2019/">Amazon re:MARS 2019 in Las Vegas, Nevada</a>” presentation. He gave it as an example of AI, but I think it’s really an example of IA. <em>Radiologists do a lot of things. They read x-ray images, they also consult with patients, they do surgical planning, and mentor younger doctors... out of all of these tasks, one of them seems amenable to AI automation or acceleration -- that's why many teams including mine are building systems like these to have AI enter this task of reading x-ray images. </em> <blockquote class="twitter-tweet"> <p dir="ltr" lang="en">2016: <a href="https://twitter.com/geoffreyhinton?ref_src=twsrc%5Etfw">@GeoffreyHinton</a> says we should stop training radiologists, because radiologists will soon be replaced by deep learning</p> 2019: There is a shortage of radiologists. # replaced ≈ 0 What did Hinton miss? by <a href="https://twitter.com/GaryMarcus?ref_src=twsrc%5Etfw">@garymarcus</a> & <a href="https://twitter.com/MaxALittle?ref_src=twsrc%5Etfw">@MaxALittle</a> <a href="https://t.co/rxTTNxaeBN">https://t.co/rxTTNxaeBN</a> — Gary Marcus (@GaryMarcus) <a href="https://twitter.com/GaryMarcus/status/1186980951643099136?ref_src=twsrc%5Etfw">October 23, 2019</a></blockquote> <script async src="https://platform.twitter.com/widgets.js" charset="utf-8"></script> Does anyone really want to <b>replace</b> radiologists with machines at this time? With human lives at stake? Probably not. But a machine-assisted radiologist? That is interesting. After the first versions are released, radiologists can work with engineers to teach the machines to do a better job in finding problems. And eventually there can be a feature set that allows the radiologists to teach the machines directly, without the constant participation of the engineers. And training data from all over the world can be shared to further optimize results. <h2>When is IA superior? </h2> <img class="wp-image-3027 size-medium" src="https://wordpress.appsilon.com/wp-content/uploads/2019/10/love-death-robots-cms-still-600x400.jpg" alt="The pilot works well with the ship's AI in Netflix short Lucky 13 from the Love Death Robots series" width="600" height="400" /> Another beneficial human and machine partnership depicted in “<a href="https://www.imdb.com/title/tt9788508/">Lucky 13</a>.” In short, IA is superior when… ...only a limited amount of labeled data, or only unlabeled data exists for a given task ...a task requires empathy and//or negotiation between humans ...a task requires a notion of causality, not just correlation ...system boundaries exist. Crucial data cannot be captured by sensors. Data input doesn’t cover the entirety of the problem. ....a problem exists within a regulated environment in which machines still struggle with decisions, such as in medicine and surgery ...a task is so critical that only a human can make the final decision ...a task requires more than <a href="https://towardsdatascience.com/ai-for-everyone-what-andrew-ng-want-to-convey-with-this-non-technical-course-in-30-points-bedaea57c81b">2-3 seconds</a> of human thought The above criteria probably describe most business and research problem scenarios. Consider moving away from the approach of "how do we replace our staff with Artificial Intelligence agents," and instead move towards "what repeatable, routinizable task can we automate in order to free up time for our human teammates? How do we unleash more intuitive and brilliant ideas by increasing their bandwidth? How do we prevent problems in our facilities by partnering machines and humans?" Thanks for reading. In the next post, we'll look at "How to Implement an IA Solution." Here are the previous posts: <a href="https://appsilon.com/decision-making-support-systems-1/">What Is Artificial Intelligence?</a> <a href="https://appsilon.com/decision-making-support-systems-2/">What Is Intelligence Augmentation?</a> Follow me on Twitter <a href="https://twitter.com/_joecha_">@_joecha_</a> <h2>Follow Appsilon Data Science on Social Media</h2> Follow <a href="https://twitter.com/appsilon">@Appsilon</a> on Twitter! Follow us on <a href="https://www.linkedin.com/company/appsilon">LinkedIn</a>! Don’t forget to sign up for our <a href="https://appsilon.com/blog/">newsletter</a>. And try out our R Shiny <a href="https://appsilon.com/opensource/">open source</a> packages!
Contact us!
