Ol Pejeta Conservancy and AI - Wildlife Species Classification with Camera Traps
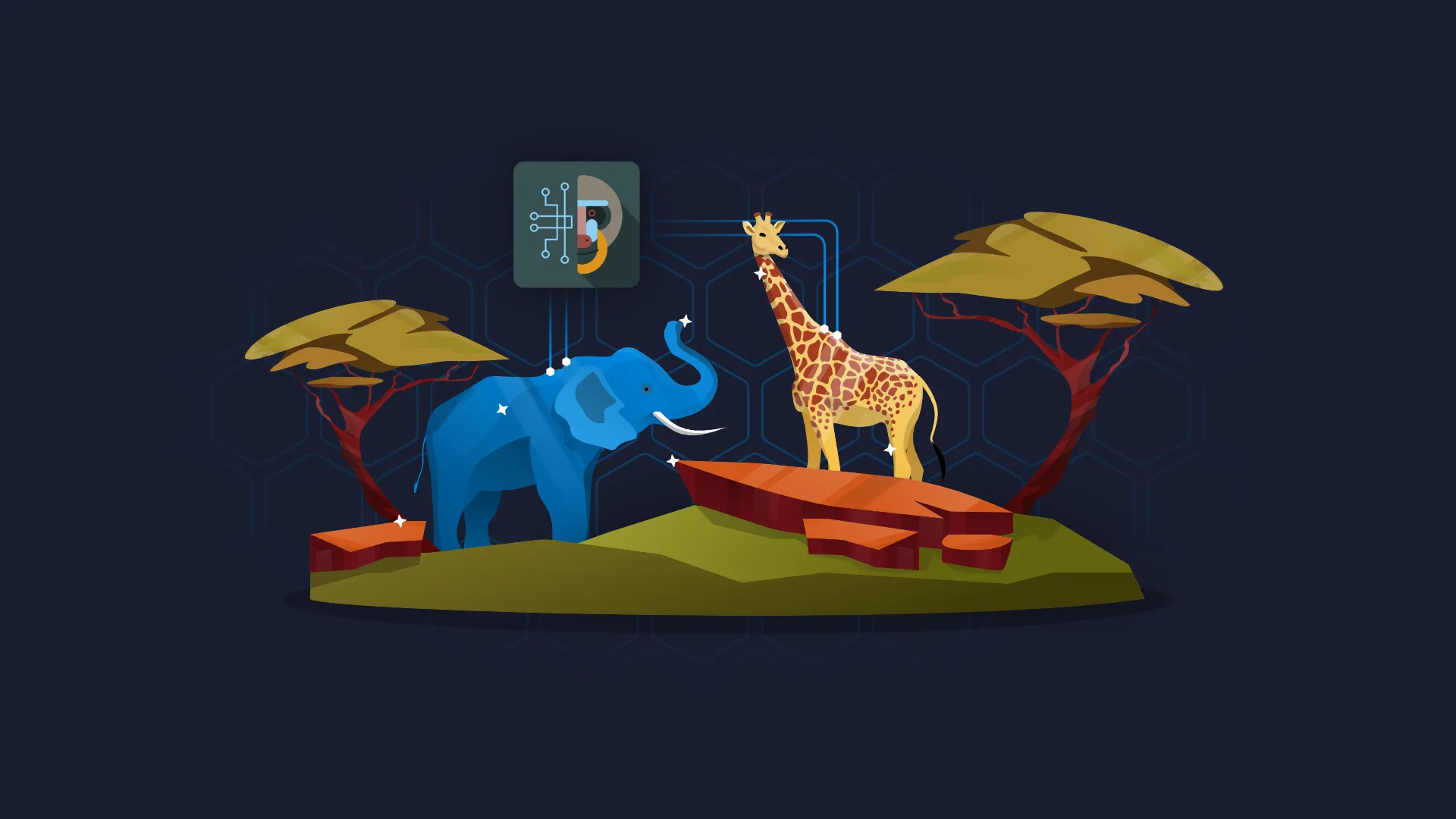
<b>AI and biodiversity</b> - two seemingly opposite topics. One is very much artificial and expanding in influence, and the other is very much organic and sadly, diminishing. Both influence us and are influenced by us, but can we apply the former to save the latter? We’ve teamed up with the Ol Pejeta Conservancy to try. We’re <b>using AI</b> to identify, <b>classify</b>, protect, and preserve <b>wildlife</b> on a massive scale.
Biodiversity is critical in supporting our health, well-being, and the planet and we rely on nature and the services it provides every day. Technology has played a key role in helping conservationists protect and preserve the natural world. Artificial Intelligence (AI) is a <a href="https://appsilon.com/applying-ai-to-nature-conservation/" target="_blank" rel="noopener">top emerging technology for biodiversity conservation</a>.
Despite successful implementation and an ever-growing potential in subfields of life sciences, using AI or computer vision is <a href="https://arxiv.org/abs/2301.02211" target="_blank" rel="nofollow noopener">rarely taught</a> to ecologists and conservationists. We think it’s time to change that.
TOC:
<ul><li><a href="#appsilonai">Appsilon's AI Solution for Wildlife Conservation</a></li><li><a href="#olpejeta">Ol Pejeta Conservancy Wildlife Imagery</a></li><li><a href="#cameratrap">Challenges of Species Identification with Camera Trap Imagery</a></li><li><a href="#automating">Automating Wildlife Image Classification with AI</a></li><li><a href="#results">Results of Wildlife Image Classification Models</a></li></ul>
<hr />
<h2 id="appsilonai">Appsilon's AI Solution for Wildlife Conservation</h2>
With AI, researchers can reduce the manual labor required to collect and analyze data. They can remove the tedious, time-consuming tasks and focus on identifying and solving problems.
As part of our <a href="https://appsilon.com/data-for-good/" target="_blank" rel="noopener">Data for Good initiative</a>, we promote and increase the adoption of these technologies in nature and biodiversity conservation. We aim to make AI accessible to those who can make an impact with it.
<a href="https://appsilon.com/data-for-good/mbaza-ai/" target="_blank" rel="noopener">Mbaza AI</a>, our open-source AI algorithm that allows rapid biodiversity monitoring at scale, has <a href="https://appsilon.com/mbaza-ai-update/" target="_blank" rel="noopener">established a strong presence in Gabon</a>. This flagship project was successfully deployed and continues to accelerate wildlife research in West Africa.
<h3>AI Wildlife Image Classification in the Savannah</h3>
Non-invasive technology, such as camera traps, and analytical software offer exciting opportunities for refining biodiversity monitoring methods. And they are not limited to single ecosystems. And so we’re happy to state that Mbaza AI is spreading out of the jungles of Gabon and into the plains of Kenya.
The Appsilon ML team has been working with the <a href="https://www.olpejetaconservancy.org/" target="_blank" rel="nofollow noopener">Ol Pejeta Conservancy</a> to test classifying images of plains animals using their migratory corridors. This post will introduce the dataset and initial modeling work carried out utilizing transfer learning through <a href="https://docs.fast.ai/" target="_blank" rel="nofollow noopener">fastai</a> to leverage pre-trained models.
<img class="size-full wp-image-18358" src="https://webflow-prod-assets.s3.amazonaws.com/6525256482c9e9a06c7a9d3c%2F65b01bbf4367c4d0119182db_elephant-wildlife-image-classification-with-AI-sml-re.webp" alt="An image showing a migrating elephant (Image credit: Ol Pejeta Conservancy)." width="400" height="225" /> An image showing a migrating elephant (Image credit: Ol Pejeta Conservancy).
<h2 id="olpejeta">Ol Pejeta Conservancy and Wildlife Imagery</h2>
Ol Pejeta Conservancy is over 90,000 acres in central Kenya committed to conserving biodiversity, protecting endangered species, driving economic growth and improving the lives of rural communities. It is home to a diverse population of wildlife, including eight near-threatened, five endangered, and one critically <a href="http://earthsendangered.com/search-regions3.asp" target="_blank" rel="nofollow noopener">endangered species</a>. To gain a deeper understanding of the wildlife population and their movement habits, Ol Pejeta has set up a number of camera traps across their migratory corridors that allow wildlife to travel in and out of the conservancy. These camera traps are motion-activated, taking a series of images upon detection, resulting in a huge library of over 5 million images taken from 2014 onwards, with a variety of wildlife.
<h3 id="cameratrap">Challenges of Species Identification with Camera Trap Imagery</h3>
<b>What does Ol Pejeta Conservancy want to do?</b>
<ul><li style="font-weight: 400;" aria-level="1">Classify images by species to understand migratory patterns in and out of the conservancy.</li></ul>
<b>Why is it difficult?</b>
<ul><li style="font-weight: 400;" aria-level="1">One of the major difficulties with large sets of images is the <b>manual labeling</b> required. Without AI, someone must painstakingly comb through each individual image and determine which, if any, animals are present; this is a monumental task when faced with over 5 million images!</li></ul>
<b>How Appsilon can help?</b>
<ul><li>We are data science experts with a specialty in AI Research and Computer Vision. We have successfully implemented our <a href="https://appsilon.com/mbaza-ai-update/">Mbaza AI</a> to classify large camera trap imagery datasets in a similar project.</li></ul>
<h2 id="automating">Automating Wildlife Image Classification with AI</h2>
<h3>Ol Pejeta Wildlife Camera Trap Dataset</h3>
Ol Pejeta Conservancy needed an accurate model to confidently automate the wildlife identification task; accurate models require a large training dataset of pre-labeled images. Ol Pejeta provided us with a subset of manually labeled data from 2018 with images from 9 different cameras in 2 migratory corridors. The cameras used are consistent in image size (1920x1080) and count of images taken when motion is detected (5).
https://youtu.be/lUa3Rr80Gm8
The dataset provides a large sample of various species captured traveling in and out of the conservation area.
For our initial studies, we worked with a subset of the 2018 data and selected the most prominent species:
<img class="size-full wp-image-18370" src="https://webflow-prod-assets.s3.amazonaws.com/6525256482c9e9a06c7a9d3c%2F65b01bc0700c6e7f89a952a7_Species-table-re.webp" alt="The approximate number of images for each of the 12 most common species identified in the 2018 training dataset." width="375" height="600" /> The approximate number of images for each of the 12 most common species identified in the 2018 training dataset.
The largest class is human activity: camera trap setup, maintenance of corridors, and passing vehicles.
Two key takeaways from this early stage:
<ol><li style="font-weight: 400;" aria-level="1">the dataset is imbalanced and will require attention to ensure that trained models are not biased</li><li style="font-weight: 400;" aria-level="1">some species will be harder to distinguish between than others</li></ol>
While most of the classes are somewhat unique, differentiating between certain species is not so simple. For example, it is hard to confuse a giraffe with an elephant (classes), but identifying gazelle types (species) is more challenging:
<img class="size-full wp-image-18366" src="https://webflow-prod-assets.s3.amazonaws.com/6525256482c9e9a06c7a9d3c%2F65b01bc28d7dfe95e411157a_Grant-gazelle-vs-Thomson-gazelle-for-AI-classification-re.webp" alt="Comparison of Grant's gazelle and Thomson's gazelle (Image credit: Ol Pejeta Conservancy)." width="939" height="270" /> Comparison of Grant's gazelle and Thomson's gazelle (Image credit: Ol Pejeta Conservancy).
<h3>Appsilon’s Approach to Image Classification with AI</h3>
First, we separate the dataset into training and validation subsets. We do this by location, using the images from 3 of the cameras for validation. Note that using random sampling for this would result in <i>data leakage</i>, as consecutively captured sequences would be present in both training and validation sets. Using a location-based approach not only avoids this pitfall but provides a more robust insight into the generalizability of the model, since the validation environment is isolated from that of the training set.
To deal with class imbalances we use a sampling approach. In the training dataset, this is sampling with replacement; each class is sampled <i>N</i> times, meaning classes with less than <i>N</i> images have multiple copies of images passed to the model. We choose <i>N</i> to be close to the median class count. To diversify these repeated images and improve the model’s generalization capability, we use the default image augmentation techniques in fastai.
For validation, we downsample the most populated classes to avoid an overly-skewed dataset, but we do not upsample the least populated classes.
Our initial modeling uses image classification techniques to produce a single label for a given image:
<img class="size-full wp-image-18364" src="https://webflow-prod-assets.s3.amazonaws.com/6525256482c9e9a06c7a9d3c%2F65b01bc200b05468d71953da_Giraffe-vs-elephant-for-AI-classification-re.webp" alt="Comparison of a giraffe and elephant classification (Image credit: Ol Pejeta Conservancy)." width="942" height="268" /> Comparison of a giraffe and elephant classification (Image credit: Ol Pejeta Conservancy).
Note that the model does not make a distinction between one or multiple animals, it simply outputs a single prediction for the full image.
<h4>Camera Trap Sequence Problem</h4>
One immediate problem that arises with this approach is that not all images contain an animal. A sequence of 5 images is taken once motion is detected, regardless of whether that motion persists for the full duration of the sequence. An example of this is shown below, where the lion is completely absent from the frame by the 4th image.
This is confusing for the model, as many images labeled as a particular species contained no animal, forcing the model to attempt to learn features in the background as features of a particular species! This behavior is of course not desirable, and so we opted to train the model on a sequence-by-sequence basis, rather than image-by-image. In the above example, this would result in a prediction akin to “this is a <i>sequence</i> of a lion.”
For the time being, we do this by choosing a single image from each sequence - one of the first three images - as we assume it becomes more likely for the animal to leave the frame as the sequence continues.
<h3>Models</h3>
We used two models for initial training: <b>ResNet50</b> and <b>ConvNeXt tiny</b>, which is the closest comparison to ResNet50’s number of parameters.
ResNet is a standard choice for a baseline model which represented a step change in the computer vision field. The 50-layer variant is almost as quick to train as the original 34-layer model due to the addition of bottleneck residual layers.
ConvNeXt is a recent innovation that uses many of the lessons learned in the recent surge of vision transformers to produce a convolutional neural network capable of matching or surpassing the accuracy achieved by vision transformers.
Both models train via transfer learning using the Adam optimizer for 5 epochs, with an initial learning rate defined by <a href="https://docs.fast.ai/callback.schedule.html#learner.lr_find" target="_blank" rel="nofollow noopener">fastai’s learning rate finder</a>.
<h2 id="results">Results of the Ol Pejeta Wildlife Image Classification Models</h2>
Both models produced promising initial results for the 12 most common species, with ResNet50 achieving 70% accuracy on the validation set, and ConvNeXt achieving 75% accuracy. The confusion matrix for the ConvNeXt model is shown below:
<img class="size-full wp-image-18352" src="https://webflow-prod-assets.s3.amazonaws.com/6525256482c9e9a06c7a9d3c%2F65b01bc410fa9efb4cc89803_AI-wildlife-species-classification-confusion-matrix-re.webp" alt="ConvNeXt model normalized confusion matrix, showing a summary of prediction results versus labels. A higher value for each matching pair (e.g. zebra - zebra) means higher accuracy of the model." width="887" height="909" /> ConvNeXt model normalized confusion matrix, showing a summary of prediction results versus labels. A higher value for each matching pair (e.g. zebra - zebra) means higher accuracy of the model.
As expected, there are some issues distinguishing between gazelles, with 24% of Thomson’s gazelles predicted as Grant’s. There are some surprising mixups between species, such as 24% of baboons confused with humans, and 17% of buffalo confused with hyenas!
<h3>Effects of Human Errors in AI Wildlife Image Classifications</h3>
Inspection of these confusions showed the model was in fact confident <i>and correct</i> on many occasions, where the predicted animal was present in the image and the labeled species was not.
<b>Manual labeling errors</b> such as these are expected in large datasets, but too many of them will result in a model that struggles to generalize.
Further difficulties arise when multiple species are present within an image, such as the examples below:
<img class="size-full wp-image-18362" src="https://webflow-prod-assets.s3.amazonaws.com/6525256482c9e9a06c7a9d3c%2F65b01bc4569a8a6eb8925b83_gazelle-in-foreground-and-zebra-in-background-for-AI-wildlife-classification-re.webp" alt="Grant’s gazelle (human label) with zebra in the background (Image credit: Ol Pejeta Conservancy)." width="1600" height="900" /> Grant’s gazelle (human label) with zebra in the background (Image credit: Ol Pejeta Conservancy).
<img class="size-full wp-image-18360" src="https://webflow-prod-assets.s3.amazonaws.com/6525256482c9e9a06c7a9d3c%2F65b01bc6ee976c4ea98f2e92_gazelle-in-foreground-and-giraffe-in-background-wildlife-image-classification-re.webp" alt="Giraffe (human label) in the background with Thomson’s gazelle in the foreground (Image credit: Ol Pejeta Conservancy)." width="1600" height="900" /> Giraffe (human label) in the background with Thomson’s gazelle in the foreground (Image credit: Ol Pejeta Conservancy).
In the first example, the model will likely predict the species correctly due to the prominence of the gazelle in the frame, but due to the single-label nature of the model, the zebra will be missed.
The second example poses a much more confusing case to the model, where the labeled species is in the background, but a gazelle has leaped onto the scene! It is likely that the model will predict this image as a gazelle, which will cause the model to try and learn from this “mistake.”
<h2>Next Steps for Ol Pejeta's Wildlife Camera AI</h2>
A number of improvements will be considered going forward, including:
<ul><li style="font-weight: 400;" aria-level="1">introducing more species into the model</li><li style="font-weight: 400;" aria-level="1">using model predictions to aid in relabeling data</li><li style="font-weight: 400;" aria-level="1">using a multi-label approach to handle images with multiple species present (also requires multiple labels per image)</li><li style="font-weight: 400;" aria-level="1">using full sequences as an input to the model, rather than selecting single images from each sequence OR</li><li style="font-weight: 400;" aria-level="1">using a detector as a prerequisite for excluding blank images, and training all images with a positive detection passed to the classifier</li></ul>
<h2>Ol Pejeta Conservancy and AI for Wildlife Species Classification</h2>
In this post, we introduced the importance of Ol Pejeta Conservancy’s impressive dataset in creating a species identification model. We trained ResNet50 and ConvNeXt tiny models on the 12 most common species and achieved results of 70% and 75% respectively.
Inspection of the results showed some expected classification difficulties, as well as unexpected confusion arising from mislabeled data. Future posts will expand on updated model results and provide further insights into the dataset.
We also hope to share the successes that the Ol Pejeta Conservancy achieves with the introduction of Appsilon’s AI for automated wildlife species classification. We are proud to be able to contribute to the important work the Ol Pejeta Conservancy is doing.
If your team is looking to speed up image classification, reduce your workload and save time, reach out to our <a href="https://appsilon.com/data-for-good/">Data for Good team</a>.